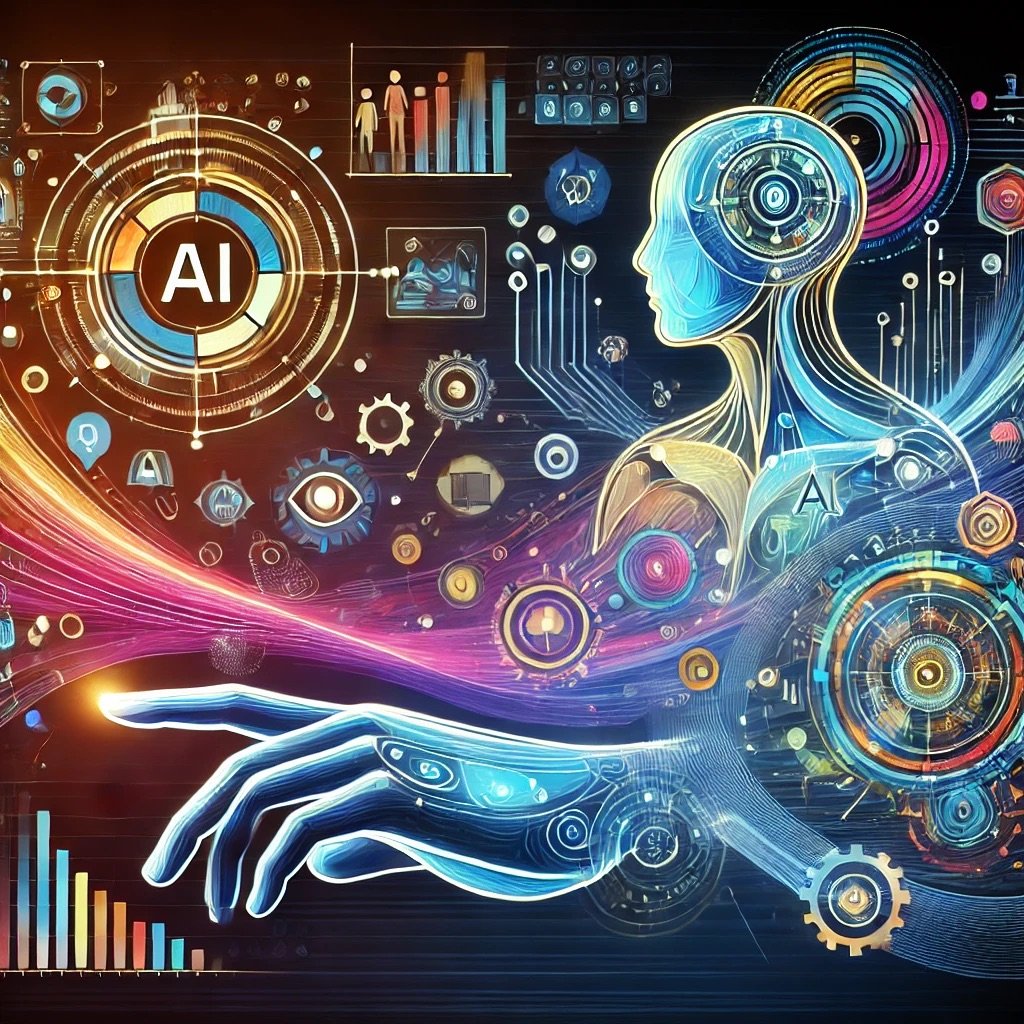
Personal Productivity Revolution: How AI Agents Are Changing Knowledge Work
Knowledge workers have long struggled with the challenges of personal productivity in an increasingly complex digital workspace. From managing overflowing inboxes to juggling multiple applications and meetings, the cognitive demands of modern work have often outpaced our capacity to manage them effectively. Agentic AI, also referred AI autonomous agents, promises to fundamentally transform how we work and help address knowledge worker productivity shortfalls.
Unlike traditional AI tools that passively respond to specific commands, AI agents can operate autonomously, make decisions, and take proactive actions on behalf of their users. These intelligent assistants enable a productivity revolution for knowledge workers by automating routine tasks, enhancing creativity, and fundamentally reshaping work processes.
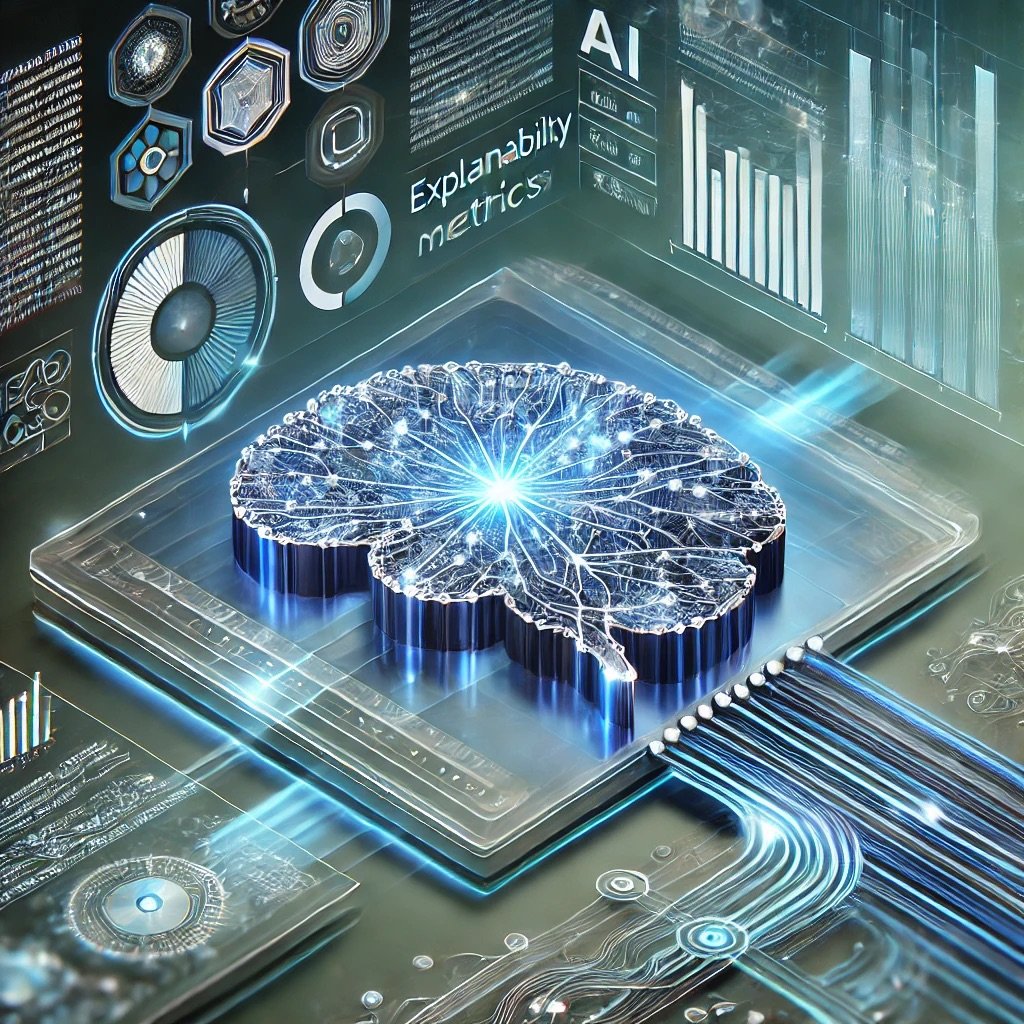
Transparency in Agent Decision-Making: Current Approaches and Challenges
Artificial intelligence (AI) agents have become integral to decision-making processes across industries and business functions—from healthcare diagnostics to financial risk assessment, customer service automation, and supply chain optimization. These agentic AI systems increasingly make or influence decisions that impact individuals' lives, organizational outcomes, and societal functions. As AI adoption accelerates, transparency in how these agents reach their decisions is a high priority and critical concern.
Transparency in AI decision-making refers to the ability to understand, interpret, and explain how AI systems arrive at specific recommendations or actions. This quality is fundamental to establishing trust, ensuring accountability, and meeting growing regulatory requirements. Without adequate transparency, organizations risk deploying "black box" systems that make consequential decisions without providing insight into their reasoning or potential biases.
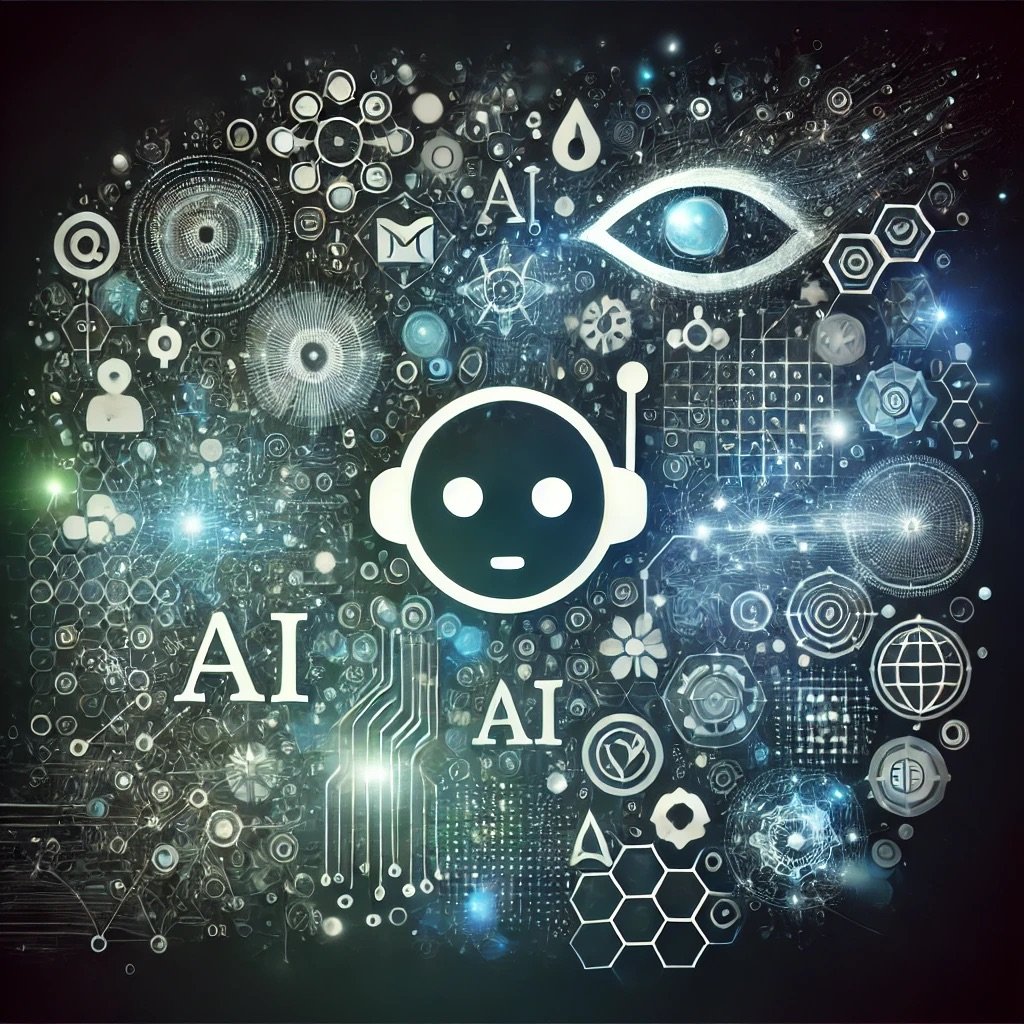
The Evolution of AI Agents: From Simple Assistants to Complex Problem Solvers
Artificial Intelligence (AI) agents are becoming an integral part of our digital landscape, evolving from rudimentary rule-following programs to sophisticated autonomous systems capable of tackling complex challenges. At their core, AI agents are software entities designed to perceive their environment, make decisions, and take actions to achieve specific goals—whether that's answering a simple question or orchestrating intricate business processes without human intervention.
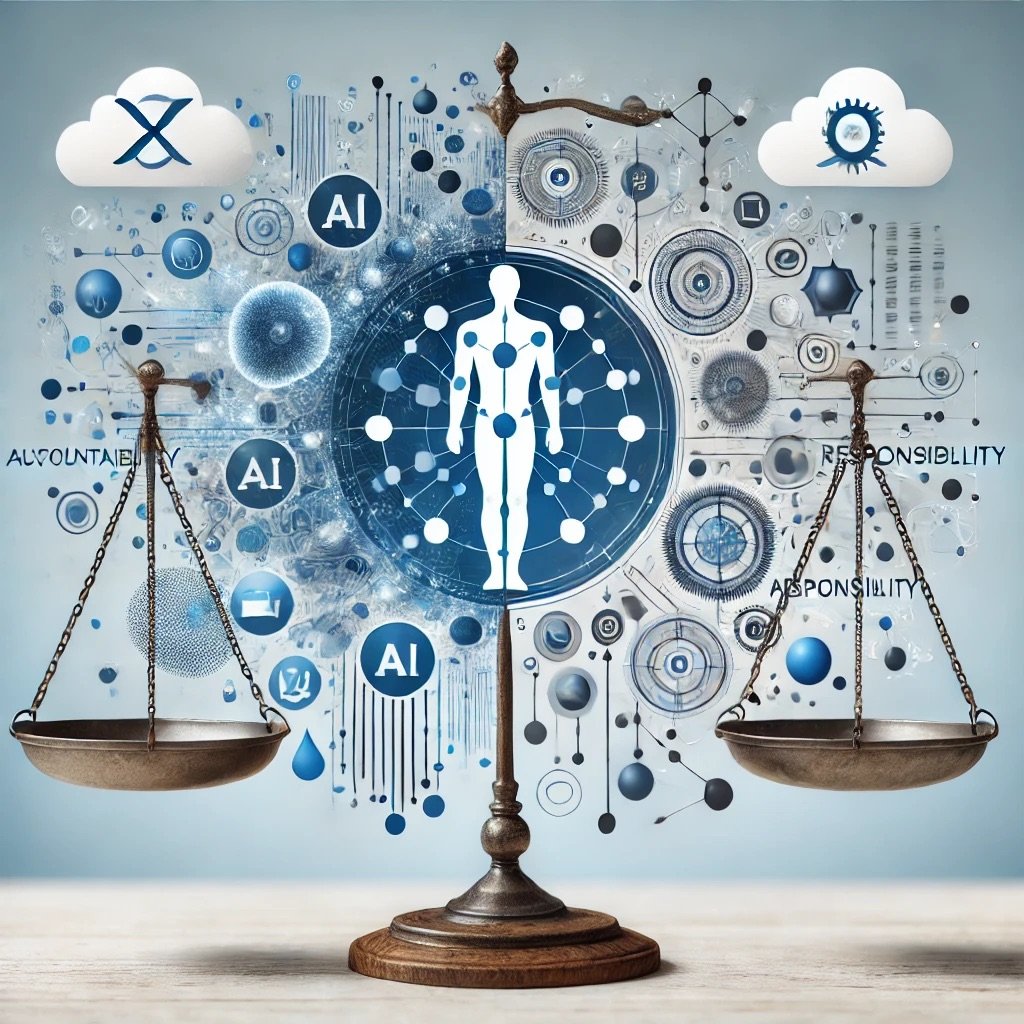
Accountability Frameworks for Autonomous AI Agents: Who's Responsible?
Agentic AI with its autonomous AI agents, is a significant advancement in artificial intelligence (AI) technology, capable of making decisions with minimal human input or oversight. Unlike traditional software tools that follow explicit programming instructions, AI agents can analyze situations, adapt to changing conditions, and take actions based on their own assessment. As more businesses embrace and deploy autonomous agents, the question of accountability and governance is becoming a high priority.
When an AI system operates with high degrees of autonomy, determining responsibility for its actions presents many challenges for traditional business legal, ethical, and organizational frameworks. If an autonomous driving system makes a decision that results in an accident, or if an AI healthcare assistant recommends an improper treatment, who bears the ultimate responsibility? The developer who created the system? The organization that deployed it? The regulatory body that permitted its use? Or should we consider the AI agent itself as a responsible entity?
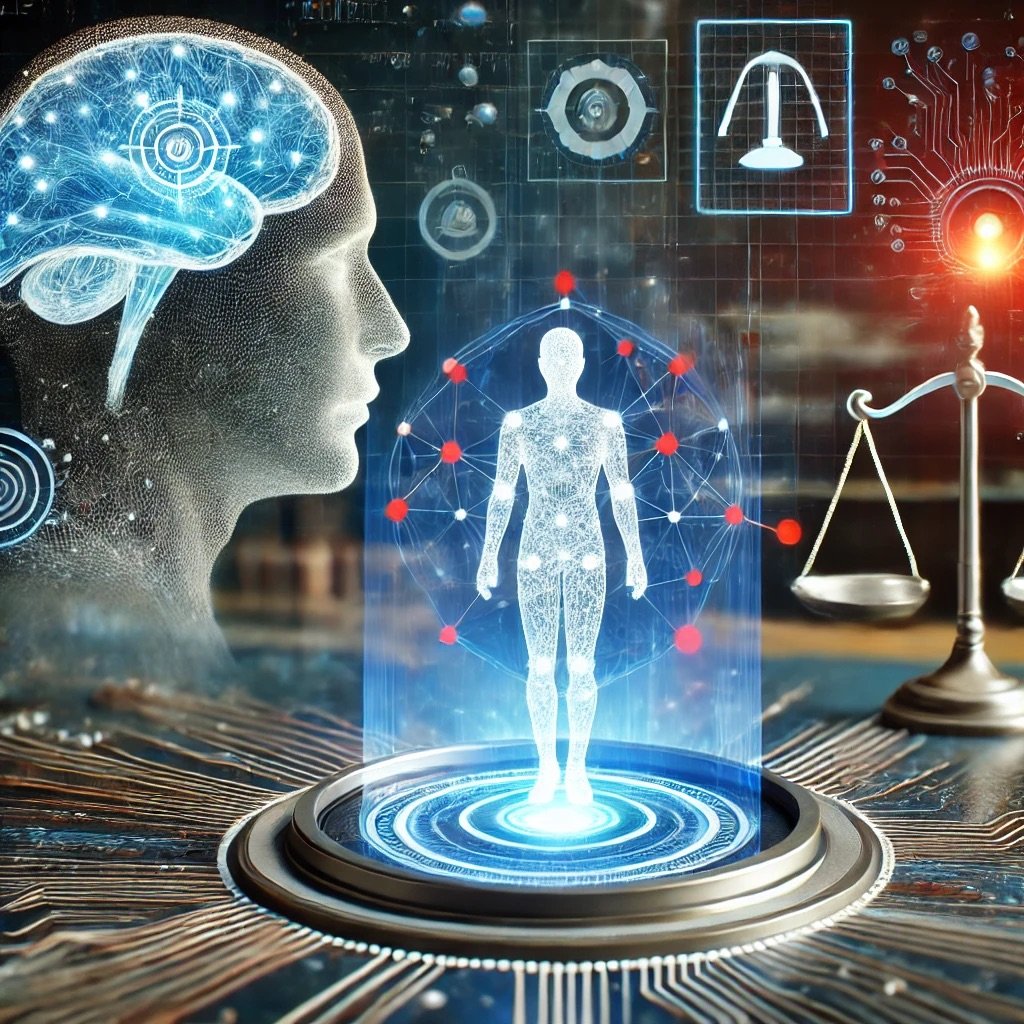
Guardrails for Agentic AI: Balancing Autonomy with Human Oversight
Agentic AI systems — autonomous artificial intelligence (AI) that can perceive, decide, and act with minimal human intervention are the latest AI technology to drive business AI transformation. As these systems become increasingly sophisticated, they promise dramatic increases in efficiency and innovation across industries. This rising autonomy though, brings legitimate concerns about reliability, ethical implications, and accountability.
Finding the optimal balance between AI autonomy and human oversight is one of the most critical challenges for businesses. Too much autonomy risks unintended consequences; too little defeats the purpose of implementation. This delicate equilibrium requires thoughtfully designed guardrails — frameworks and practices that enable AI systems to operate efficiently while ensuring human values remain at the center of their operation.
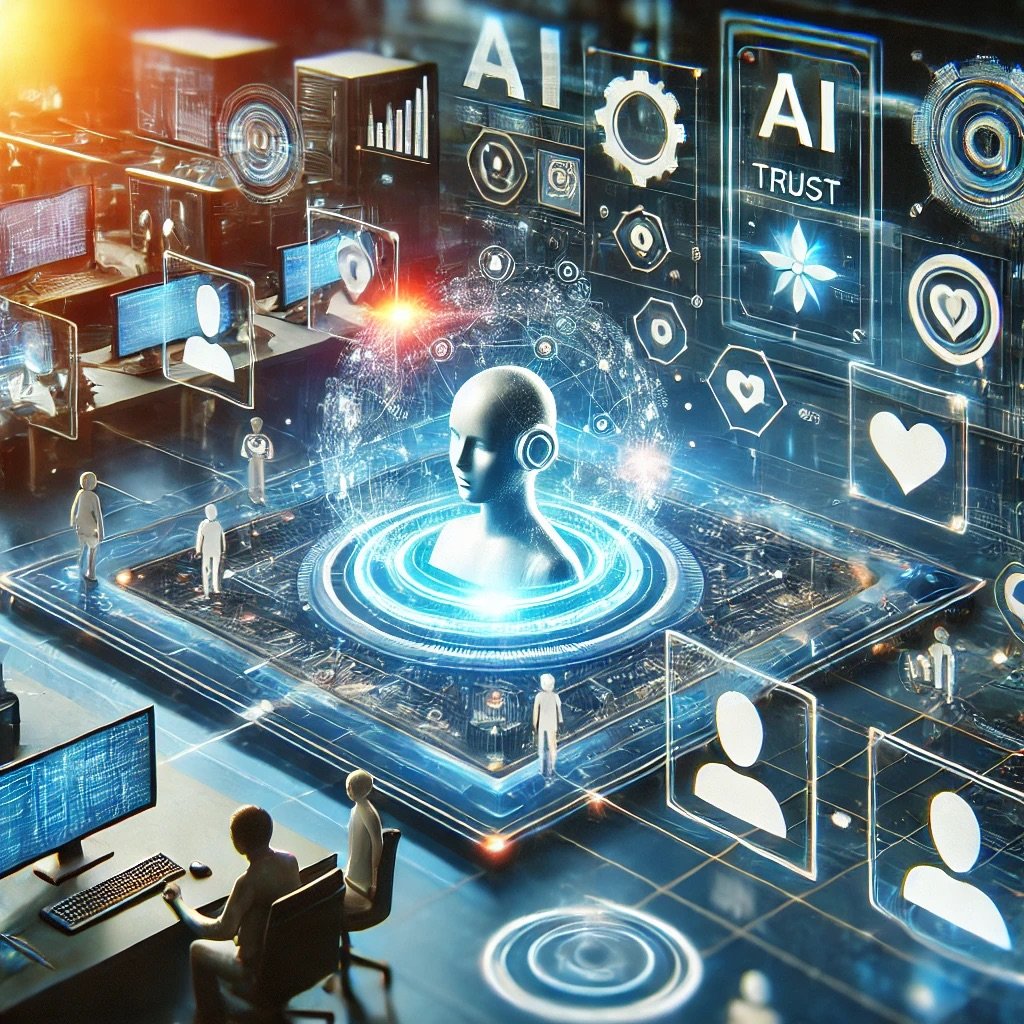
AgentExchange; “Hiring” the Digital Workforce
As we dig deeper into the business uses of Agentic AI, the simplest way to help business leaders understand how to think about autonomous agents is as a “digital workforce”. The workplace of the future, at least as we understand it today, is a collaboration between a human workforce and a digital workforce. What is agentic AI? Agentic AI is an artificial intelligence system designed to act autonomously to achieve goals, make decisions, and take actions in various environments with minimal human oversight.
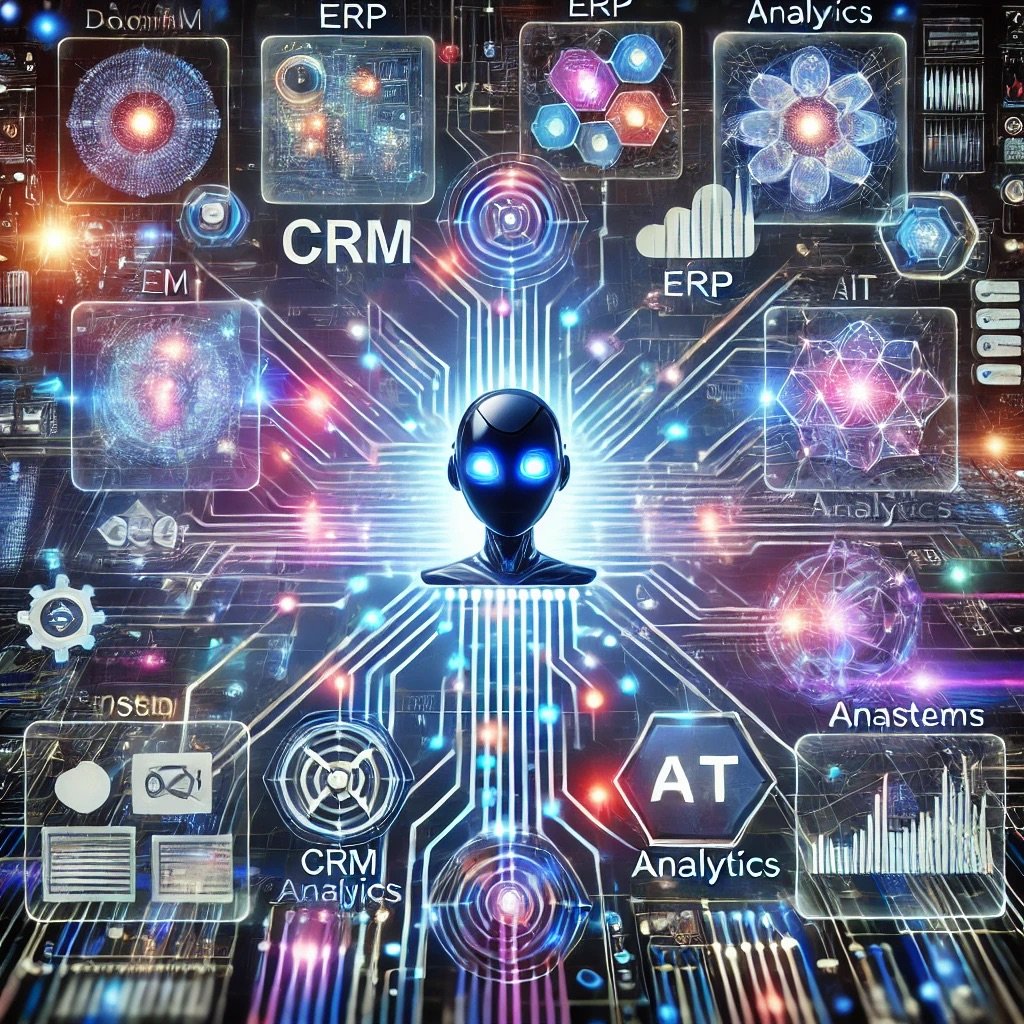
Interoperability Challenges for Agentic AI Across Platforms
Agentic AI is transforming enterprise operations by enabling autonomous decision-making, workflow automation, and intelligent orchestration across digital systems. Unlike traditional AI models, which rely on predefined inputs and outputs, agentic AI operates dynamically, responding to evolving environments and making independent decisions based on contextual data. As organizations integrate agentic AI across various business applications—CRM, ERP, service management, and analytics—interoperability has emerged as a critical challenge.
For businesses to fully leverage agentic AI, AI agents must seamlessly communicate across disparate platforms, ensuring consistency, accuracy, and efficiency. However, achieving interoperability is complex due to differences in data structures, system architectures, security requirements, and AI model variations. Let’s explore the key challenges of interoperability for agentic AI across platforms and examine potential solutions for creating a more integrated AI ecosystem.
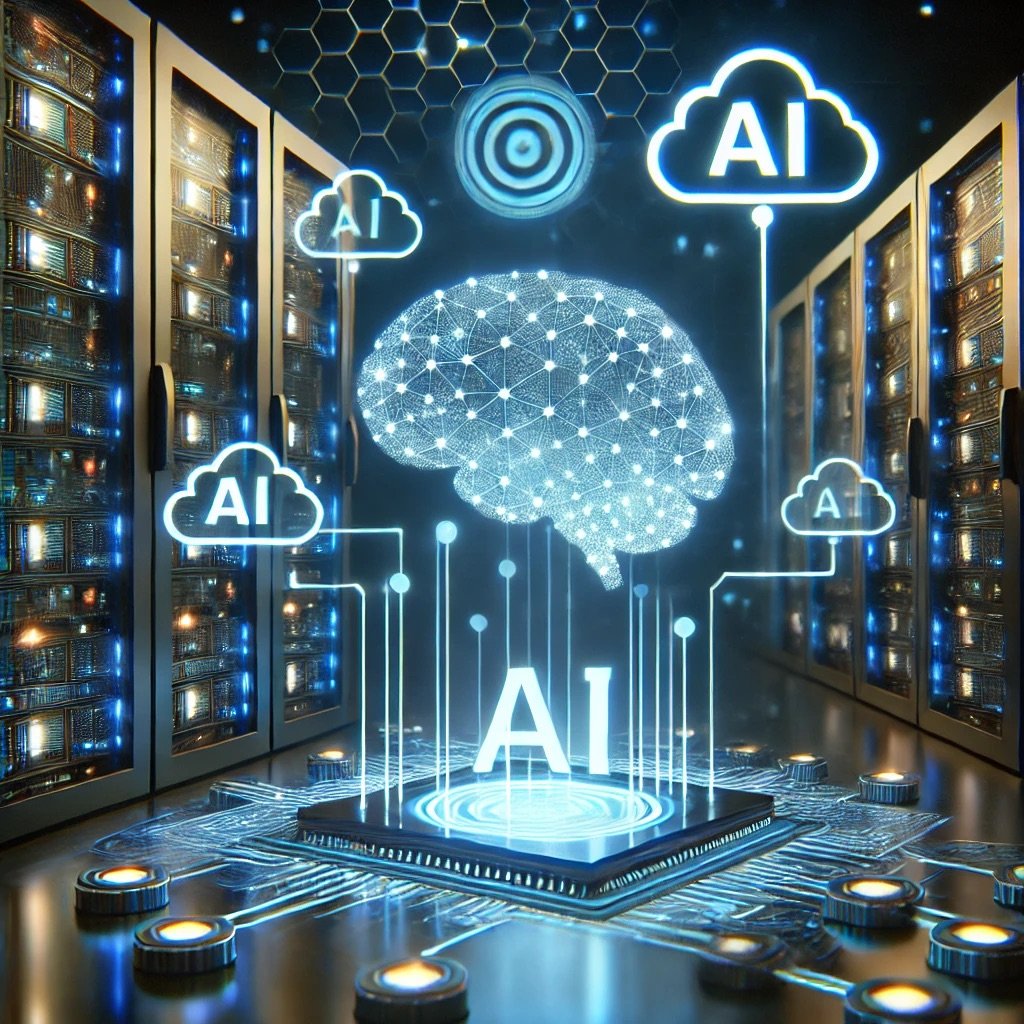
AI and Hybrid Cloud Infrastructure: Crafting a Scalable IT Strategy
Artificial intelligence (AI) is transforming how businesses approach IT infrastructure. As AI models grow in complexity and demand more computing power, organizations are moving towards hybrid and multi-cloud environments to ensure scalability, flexibility, and efficiency. This shift enables companies to optimize AI workloads while balancing cost, security, and regulatory requirements.
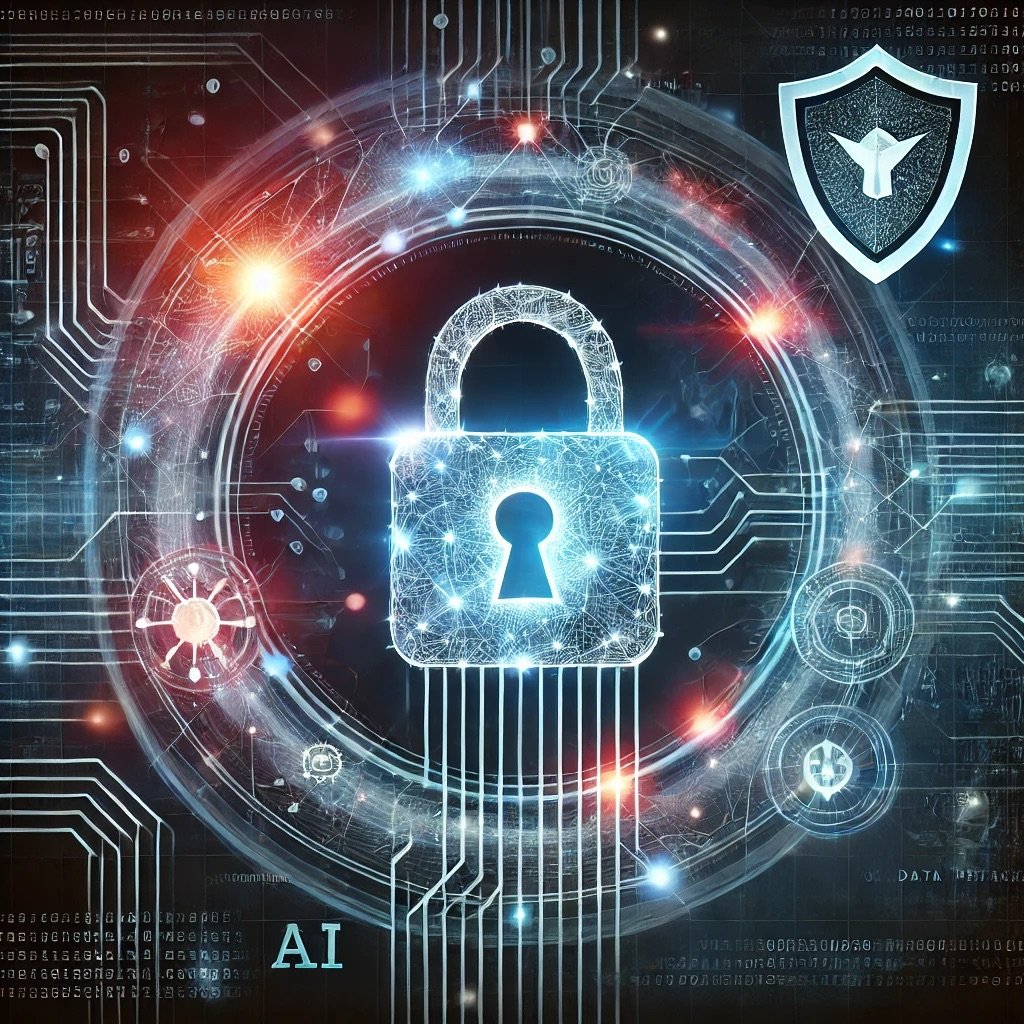
Data Privacy and AI: Balancing Innovation with IT Security in AI Strategy
Artificial Intelligence (AI) is transforming the way businesses operate, offering unprecedented capabilities in automation, decision-making, and predictive analytics. However, as AI adoption accelerates, organizations face mounting challenges in data privacy and IT security. Balancing innovation with security is a critical aspect of AI strategy, requiring a proactive approach to risk management, compliance, and ethical AI implementation.
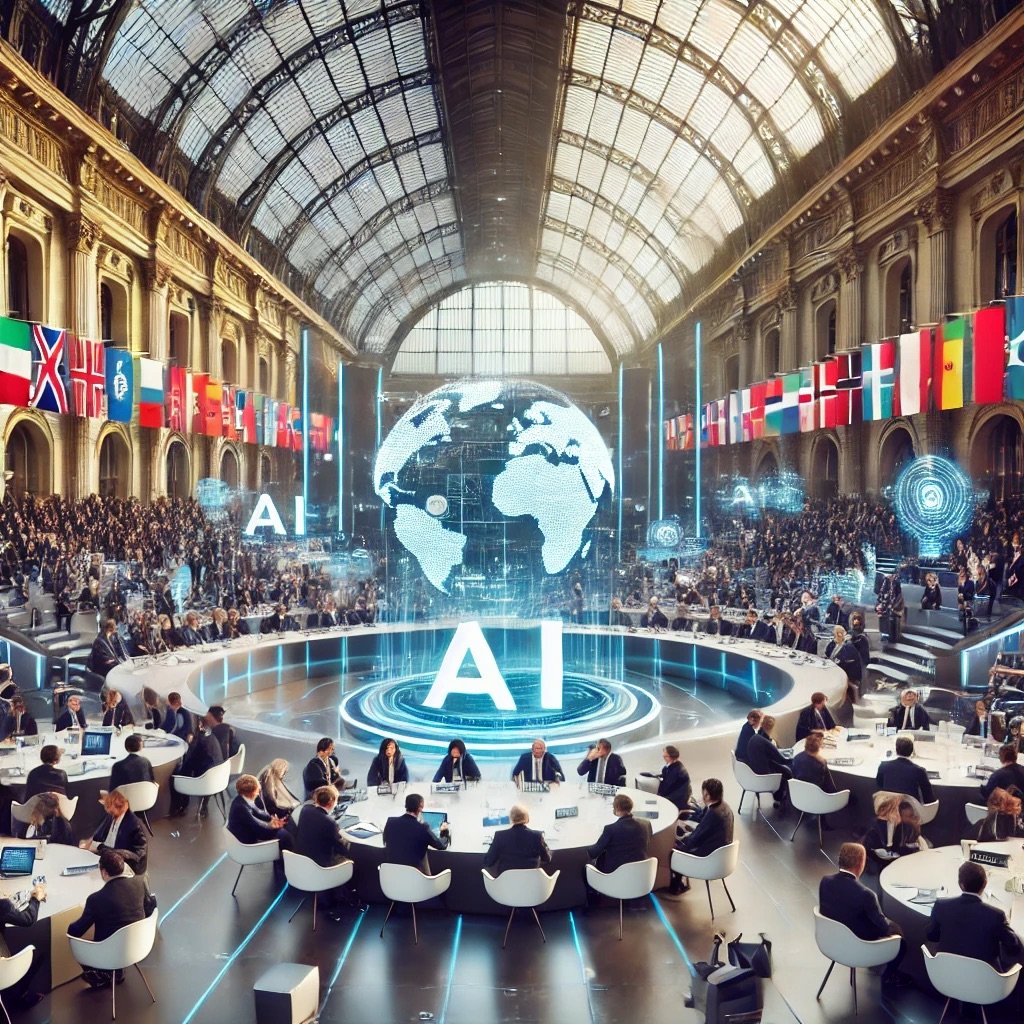
The Paris AI Action Summit 2025: A Global Gathering to Shape the Future of AI
The Paris AI Action Summit, held on February 10-11, 2025, at the Grand Palais, brought together global leaders, industry executives, and policymakers to discuss the future of artificial intelligence (AI) governance and collaboration. Co-chaired by French President Emmanuel Macron and Indian Prime Minister Narendra Modi, the summit convened representatives from over 100 countries, marking a significant step in international AI discourse.
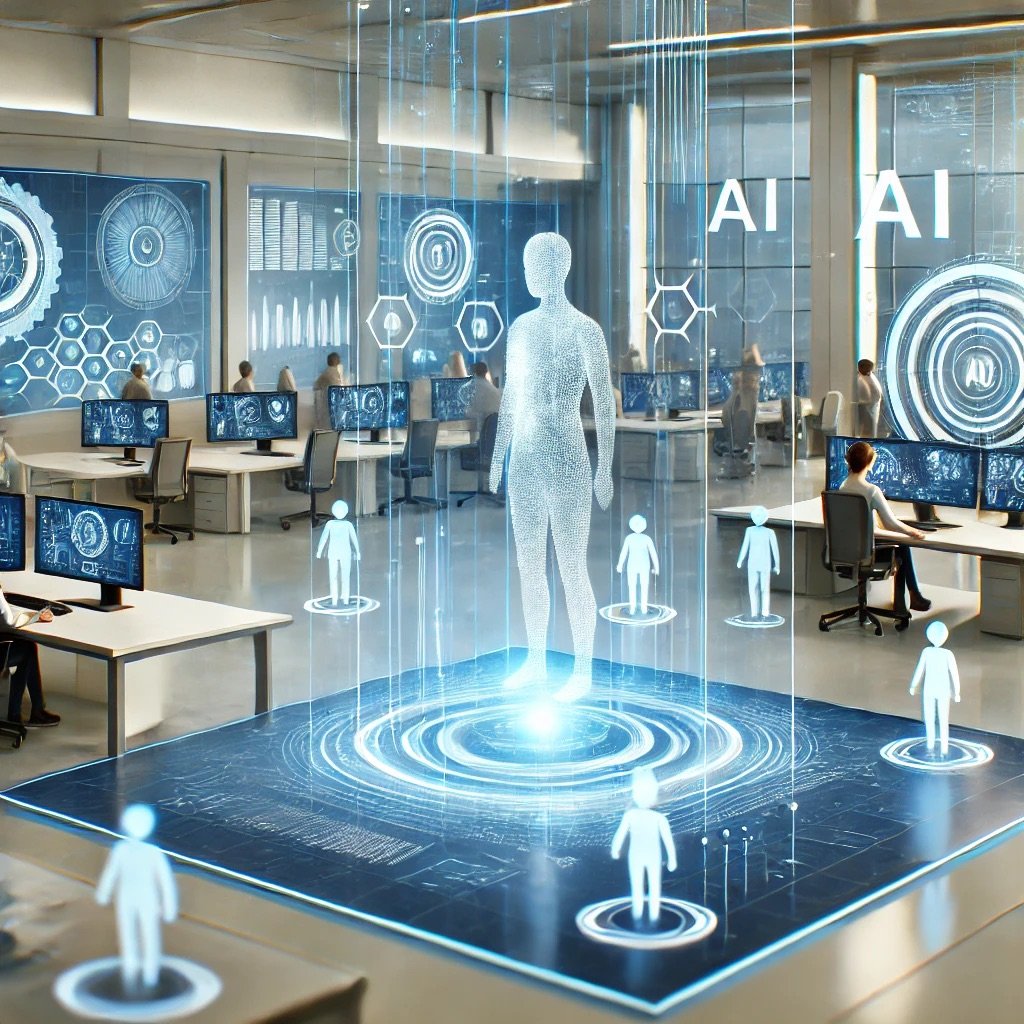
Challenges in Building Trustworthy Agentic AI Systems
Agentic AI is increasingly shaping automation and decision-making across industries, from customer service to finance and healthcare. These AI systems operate autonomously, make decisions, and interact with users and systems to execute tasks. However, building trust in agentic AI remains a significant challenge. Trustworthiness is crucial for adoption, regulatory approval, and ensuring that these systems align with human values. It’s critical for businesses implementing agentic AI to understand the key challenges in building trustworthy agentic AI systems and strategies for overcoming them.
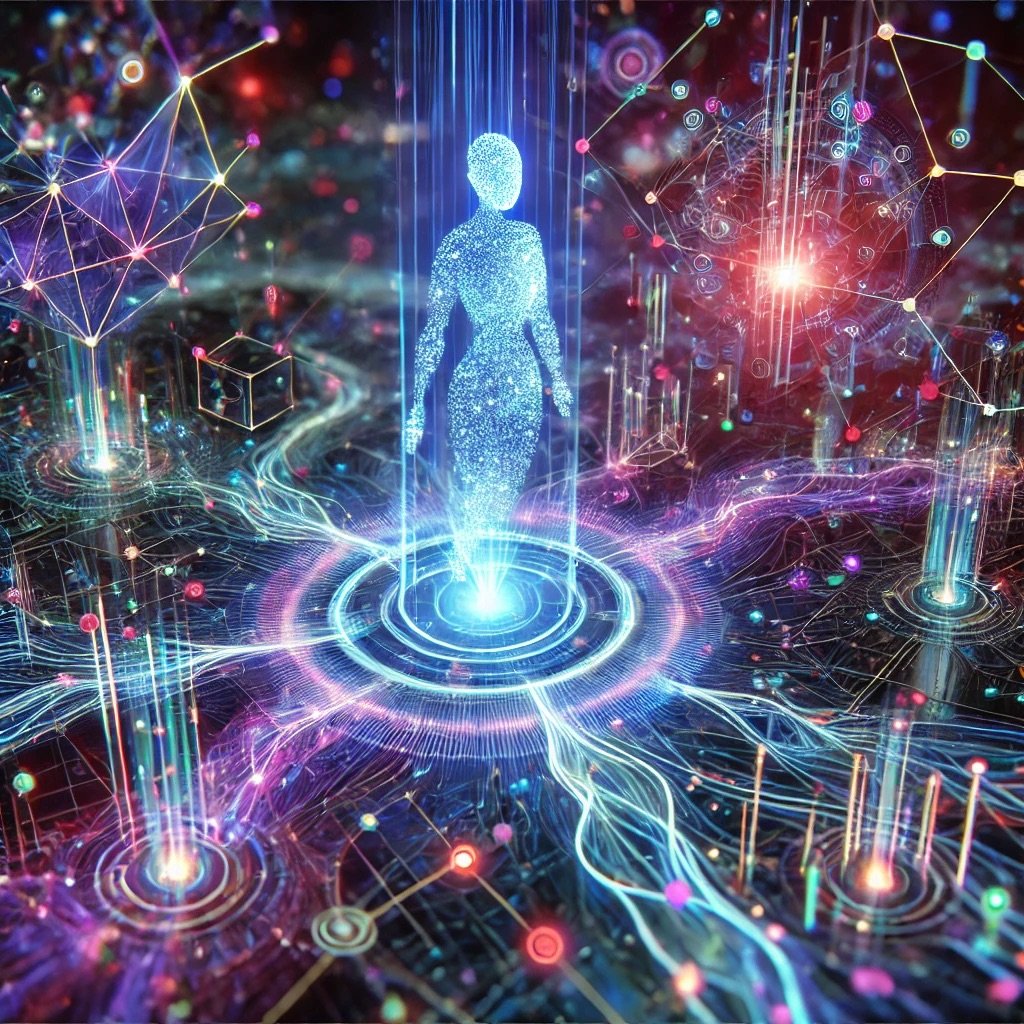
Agentic AI and the Role of Reinforcement Learning in Complex Environments
Artificial Intelligence (AI) has evolved significantly over the past few decades, with one of the most notable advancements being the development of Agentic AI—systems capable of autonomous decision-making, adaptability, and goal-directed behavior. These AI systems leverage Reinforcement Learning (RL) to navigate complex environments, learning through trial and error to optimize their performance over time. Let’s take a look at the fundamental concepts of RL, its critical role in enabling agentic AI, the challenges faced in complex environments, real-world applications, and the future of AI-driven decision-making.
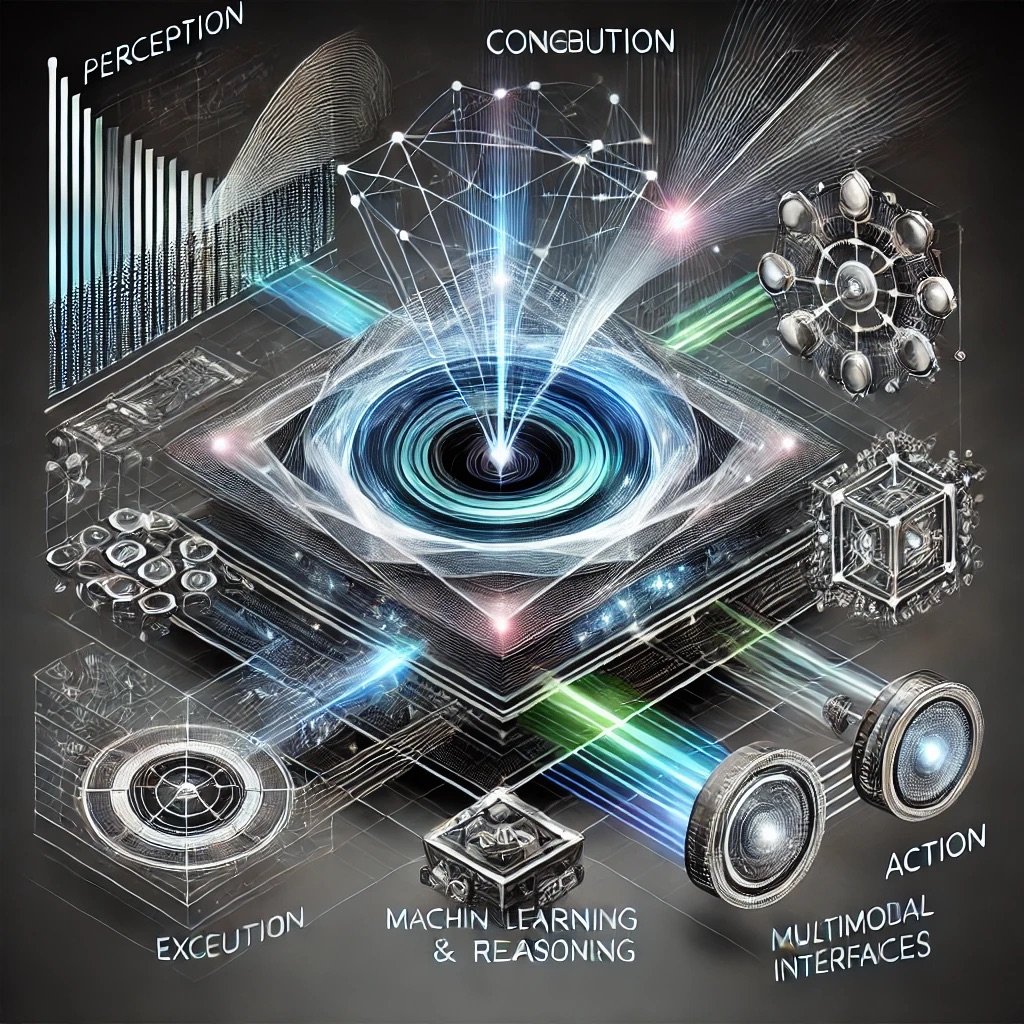
The Architecture of Agentic AI: Building Systems that Think and Act Autonomously
Agentic AI represents a significant leap in the evolution of artificial intelligence, enabling systems that can independently perceive, reason, and act in dynamic environments. Unlike traditional AI models designed for specific tasks, Agentic AI integrates advanced cognitive capabilities to perform autonomously across diverse scenarios.
The demand for such systems is growing across industries, from autonomous vehicles to smart customer service solutions. This article explores the foundational architecture of Agentic AI and the design principles critical for building systems that think and act autonomously.
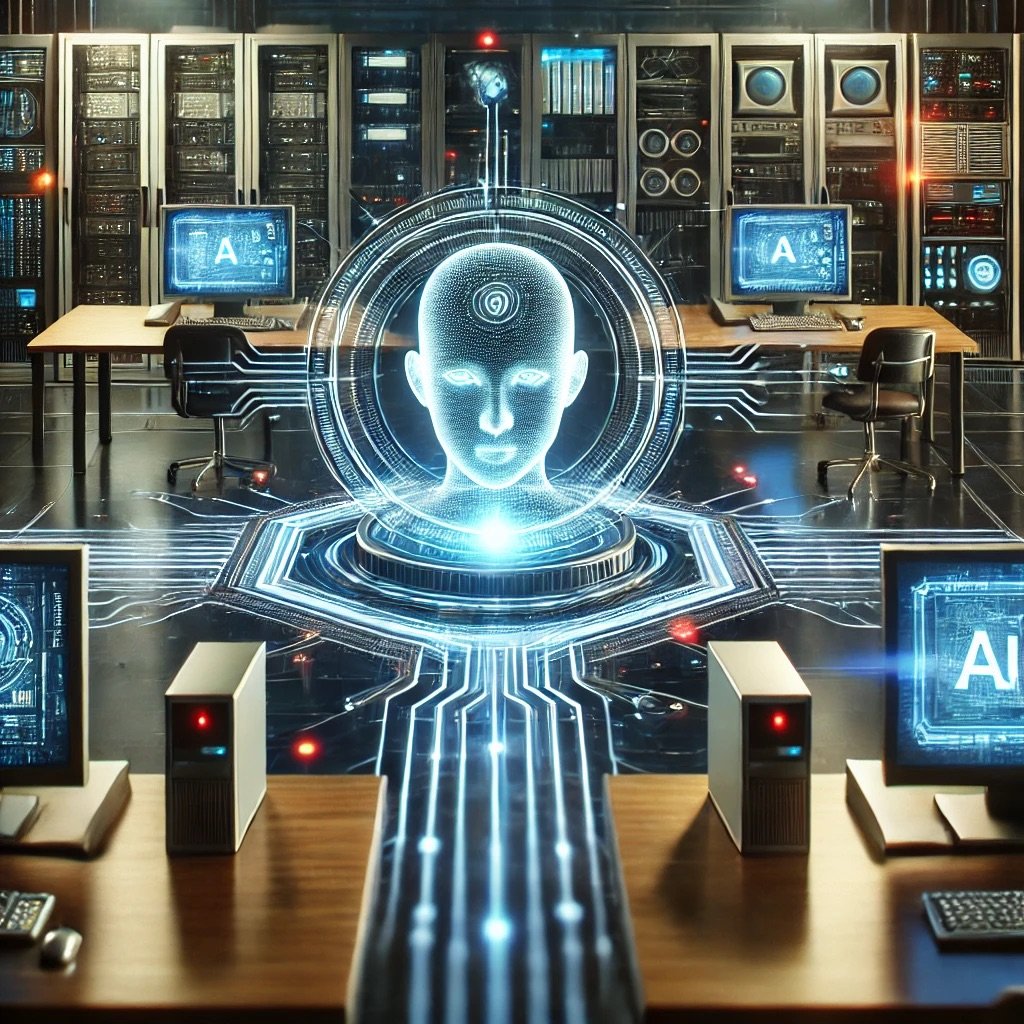
How Agentic AI Drives Innovation in Enterprise Applications
The pace of technological innovation has increased exponentially over the past few years, particularly in artificial intelligence (AI) and associated tools like language models (LMs), generative AI and the hottest new "thing", agentic AI. Enterprises are continually searching for tools and systems to transform operations supported by aging / outdated enterprise applications, to enhance their ability to innovate and stay ahead of the competition. Agentic AI has emerged as a compelling method of transformation due to its autonomous decision-making and contextual adaptability. It is redefining how businesses operate by enabling dynamic problem-solving and proactive decision-making at scale. In addition, its ability to drive innovation in enterprise applications is reshaping the future of business operations.
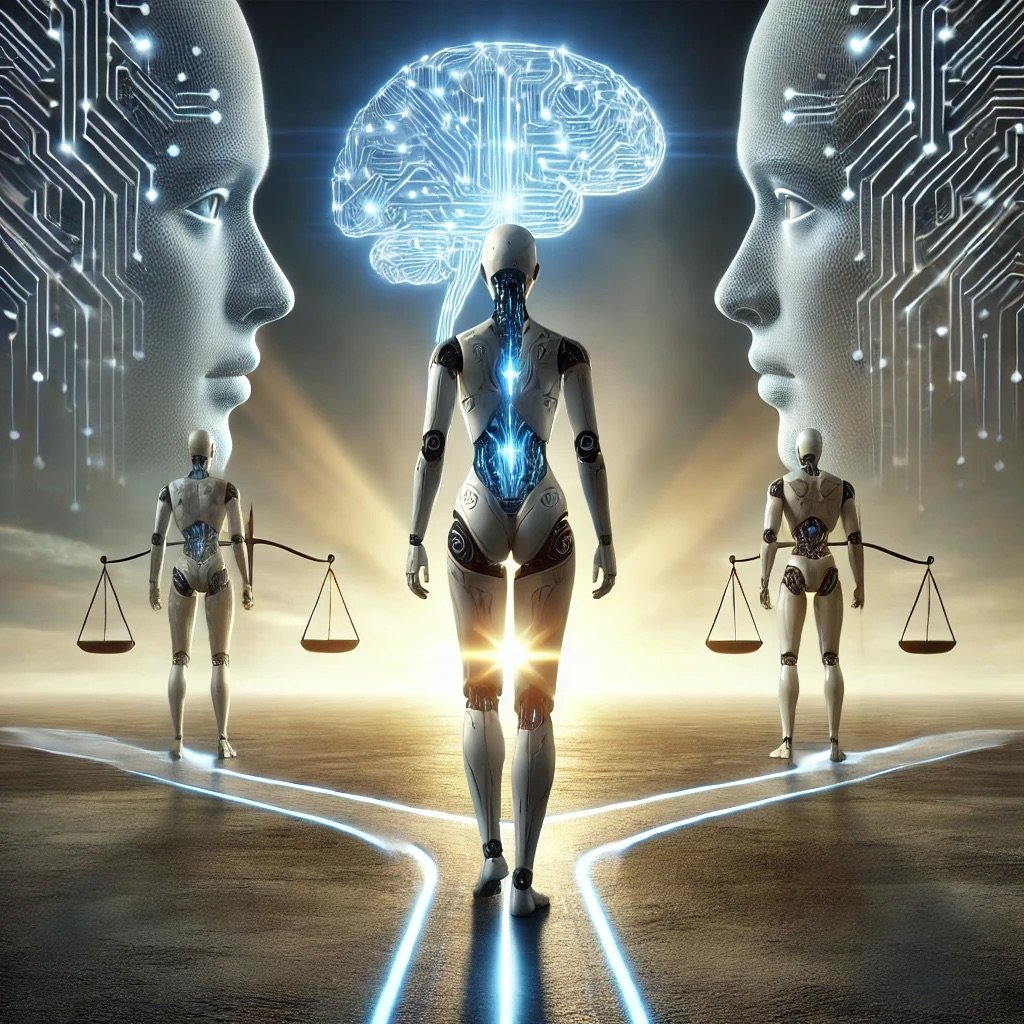
The Ethics of Agentic AI: Who is Responsible for Autonomous Decisions?
Imagine a self-driving car faced with a split-second decision: veer off the road to avoid a pedestrian but endanger its passengers or stay on course and risk the pedestrian’s life. Such scenarios were once confined to ethics classrooms, but with the rise of agentic AI, they are becoming real-world dilemmas. Agentic AI—systems capable of making autonomous decisions—is now integrated into various sectors, from healthcare to transportation. However, its growing presence raises an urgent question: who is responsible when these systems make decisions that lead to significant consequences?
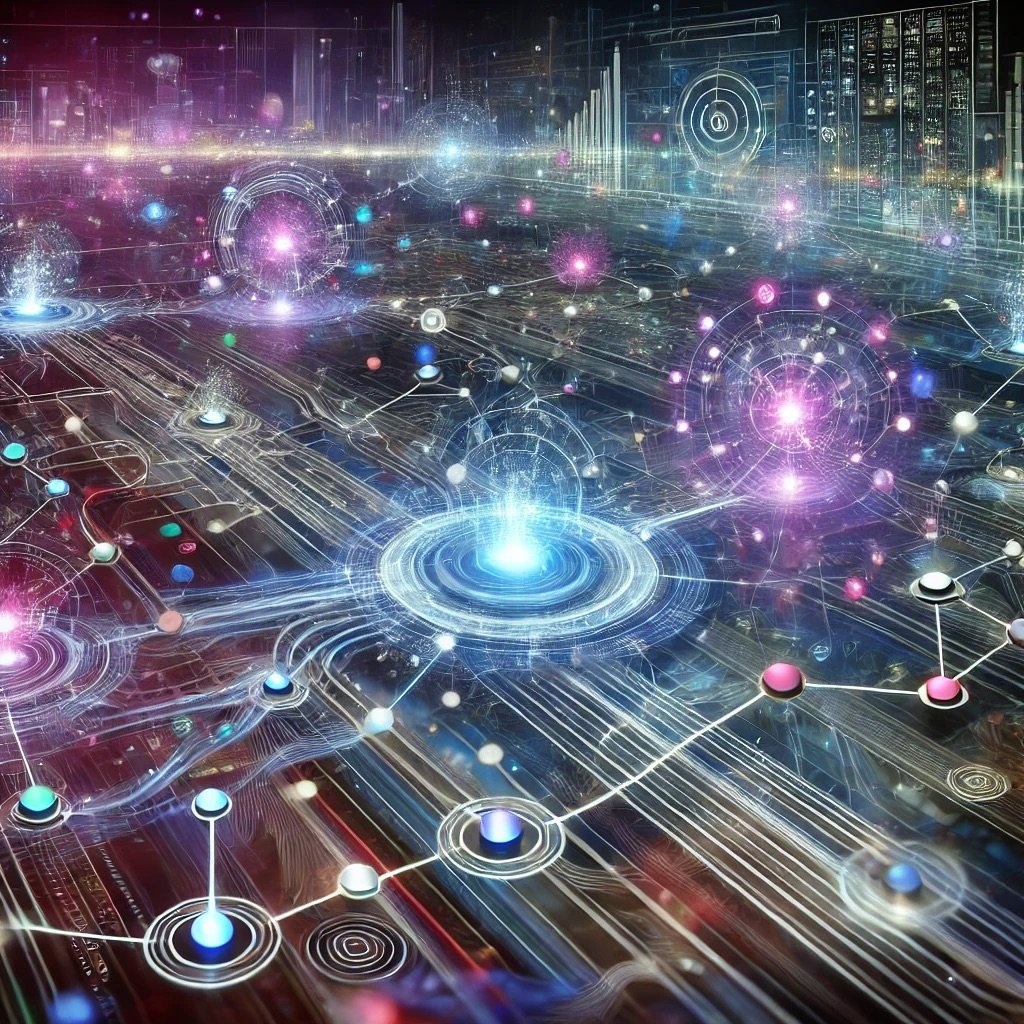
A Multi-Agent Approach to Agentic AI
Agentic AI, a concept emphasizing autonomy, goal-oriented behavior, and decision-making capabilities, is reshaping the AI landscape. Unlike traditional AI systems that heavily rely on user input, agentic AI operates independently, adapting to dynamic environments and collaborating to achieve specific objectives. By leveraging a multi-agent approach, the capabilities of agentic AI can be enhanced further, creating systems that are scalable, flexible, and efficient. Multi-agent systems (MAS) can amplify the power of agentic AI, and have a variety of high value applications across various industries and functions.
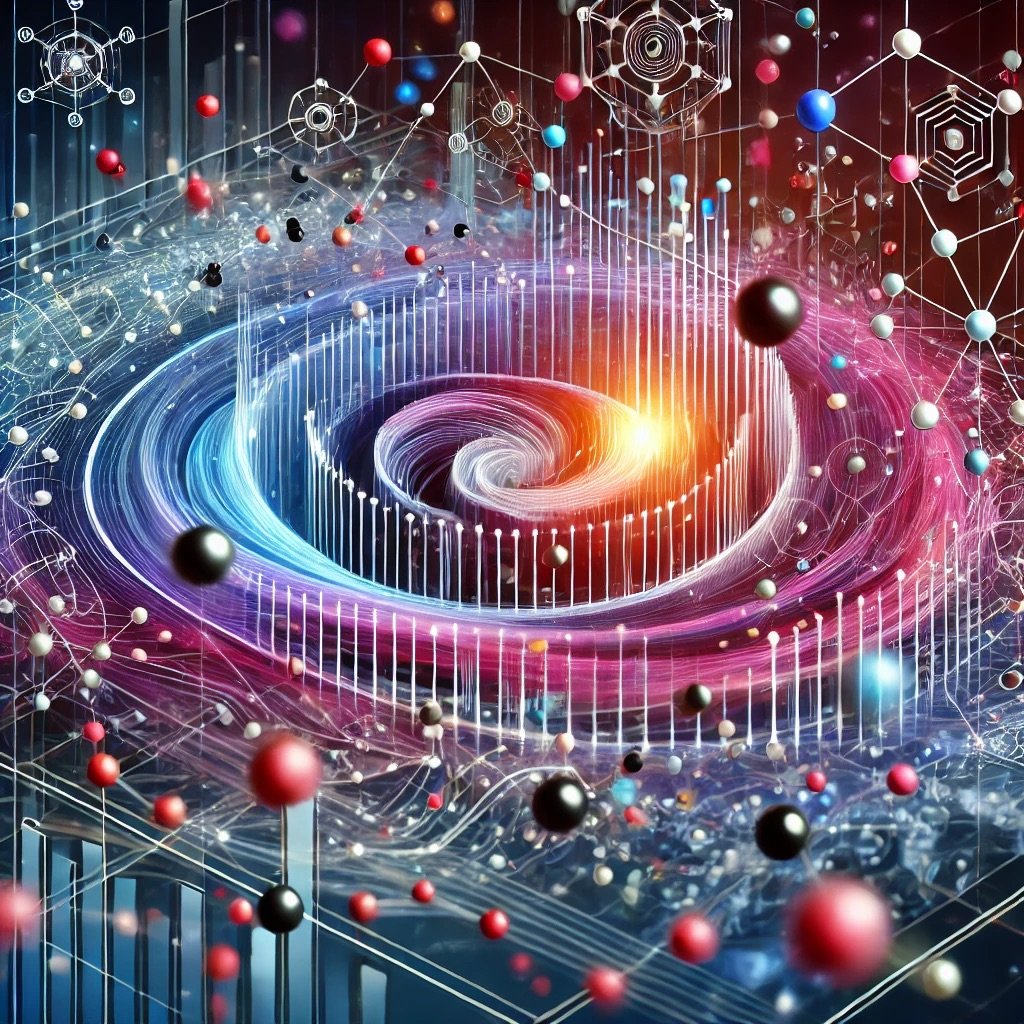
GraphRAG: The Future of Knowledge-Driven AI
The introduction of generative AI a couple of years ago created excitement, some hype and over time, a growing concern about hallucinations or inaccuracies in provided answers. Adding methods to add contextual data to the generative AI tools is proving to ground the LMs and greatly improve model accuracy, reducing or eliminating hallucinations. One of the most common frameworks is retrieval augmented generation (RAG). Advanced forms of RAG, which mash up different approaches, are being introduced, and in the proper use case, are having a large impact on business adoption of generative AI enabled tools. One of the most promising techniques to emerge is GraphRAG (Graph-based Retrieval-Augmented Generation), a cutting-edge framework that combines graph-based data structures with RAG to provide highly accurate, context-driven, and explainable AI solutions. This approach is especially impactful for tasks requiring deep reasoning over interconnected knowledge, such as complex question answering, summarization, and tailored content generation.
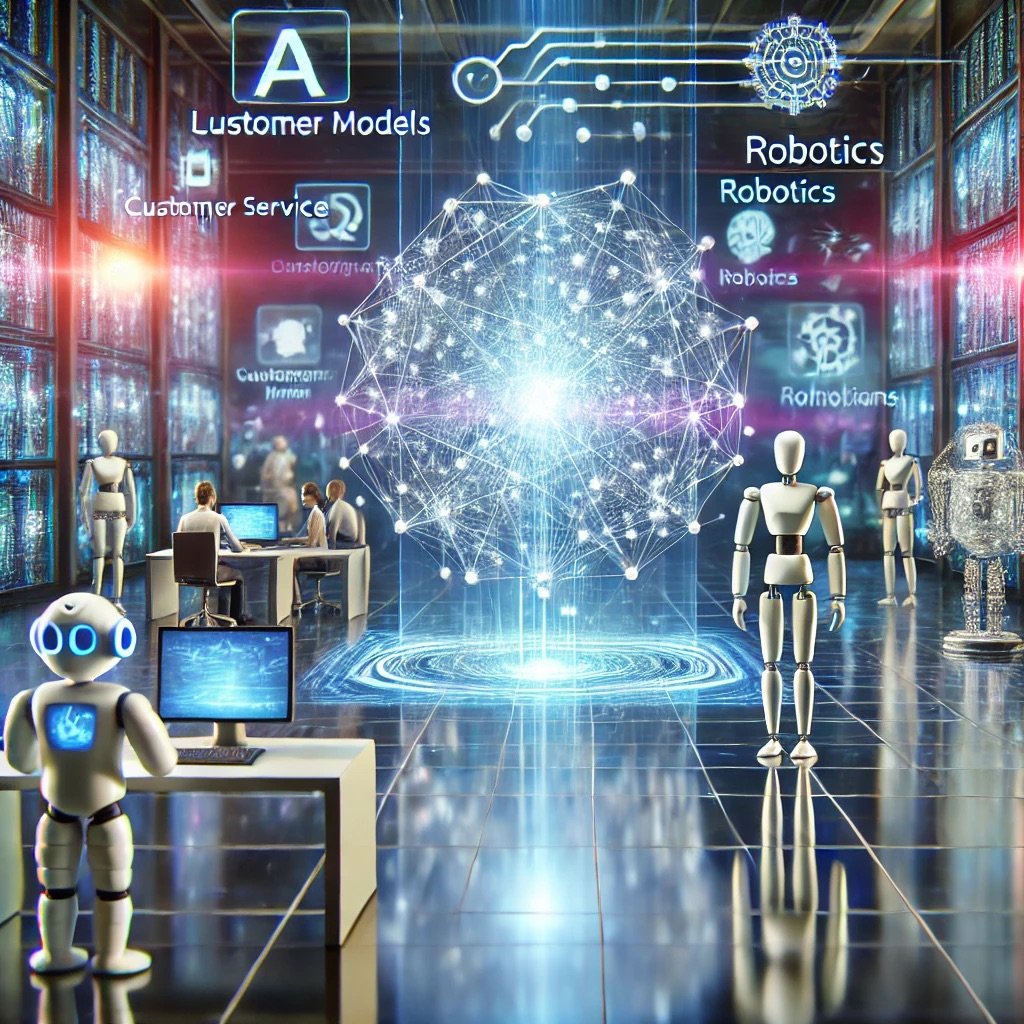
The Evolution of Language Models in 2025
Language models (LMs) are rapidly increasing in capabilities, evolving from simple statistical models to sophisticated systems capable of a growing set of use cases for business. The year 2025 is likely to be a pivotal moment in their development, with significant advancements across multiple dimensions. The various types of language models, their sizes, and their impact, including their role in enabling agentic AI, continue to expand and gain more advanced capabilities. From Large Language Models (LLMs) to Liquid Foundation Models (LFMs), these LM innovations open a great deal of value and opportunity for businesses.
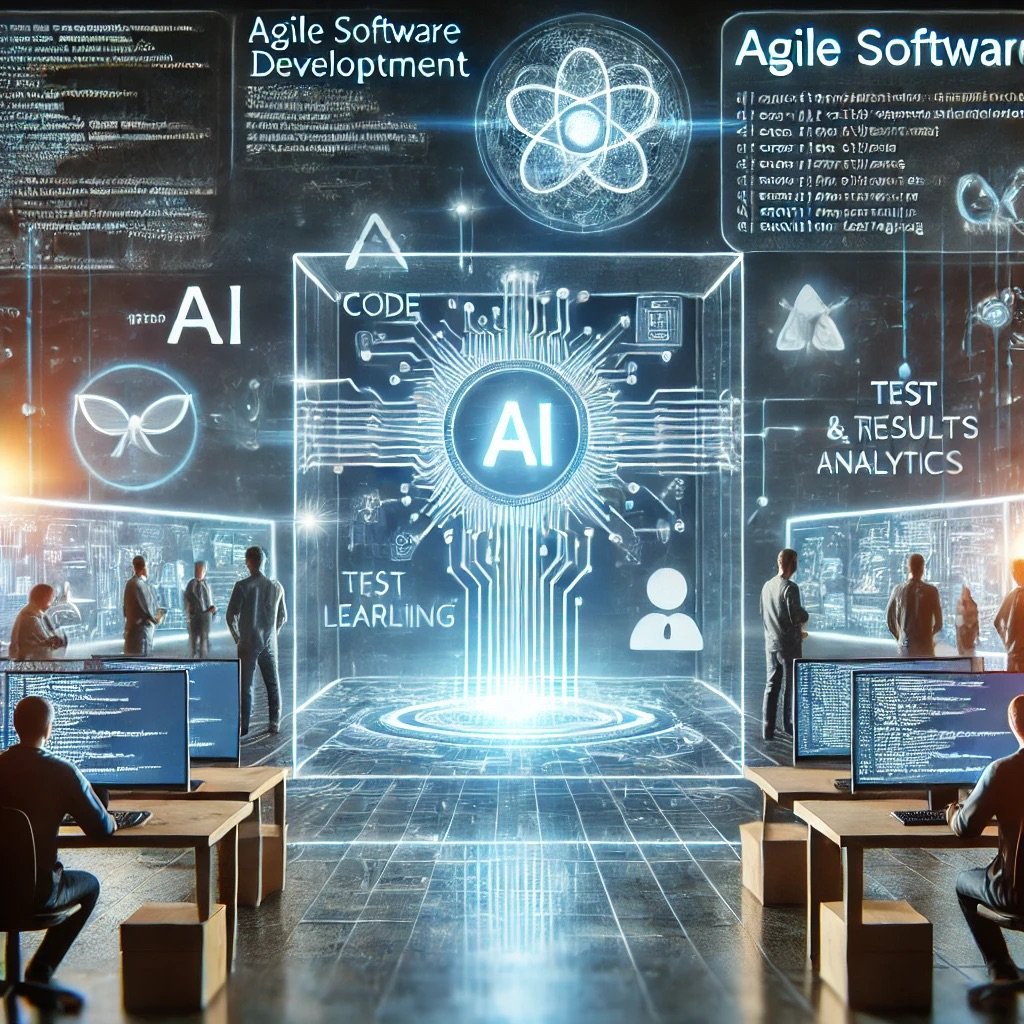
Agile Product Development with AI: Automating Code, Testing, and Deployment
Artificial Intelligence (AI) is transforming how software is developed, making it faster, smarter, and more efficient. In Agile product development, where adaptability and speed are foundational, AI is a game-changer. By applying AI to automate coding, testing, and deployment, AI tools not only streamline workflows but also empower teams to focus on innovation. The use of AI enhances the Agile software development lifecycle (SDLC) and changes the roles and necessary skills of employees.
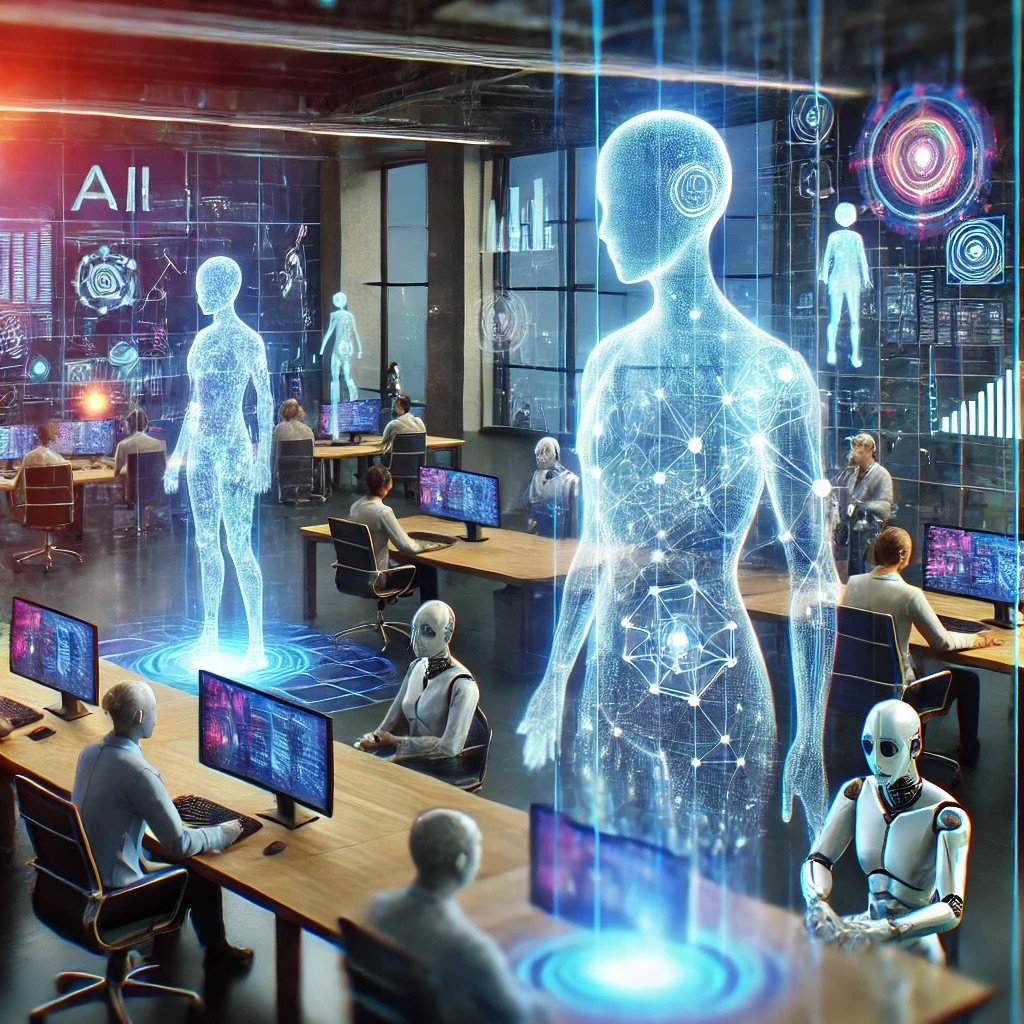
How Agentic AI is Redefining the Role of Autonomy in Digital Systems
In a business environment marked by continual rapid change, autonomy in digital systems is no longer a luxury—it’s a necessity. Business leaders are seeking smarter solutions that not only streamline operations but also proactively drive innovation and growth. Agentic AI represents a new frontier in this evolution, providing the foundation for a scalable, intelligent digital workforce. Business leaders who embrace this technology can unlock unprecedented efficiency, agility, and competitive advantage.