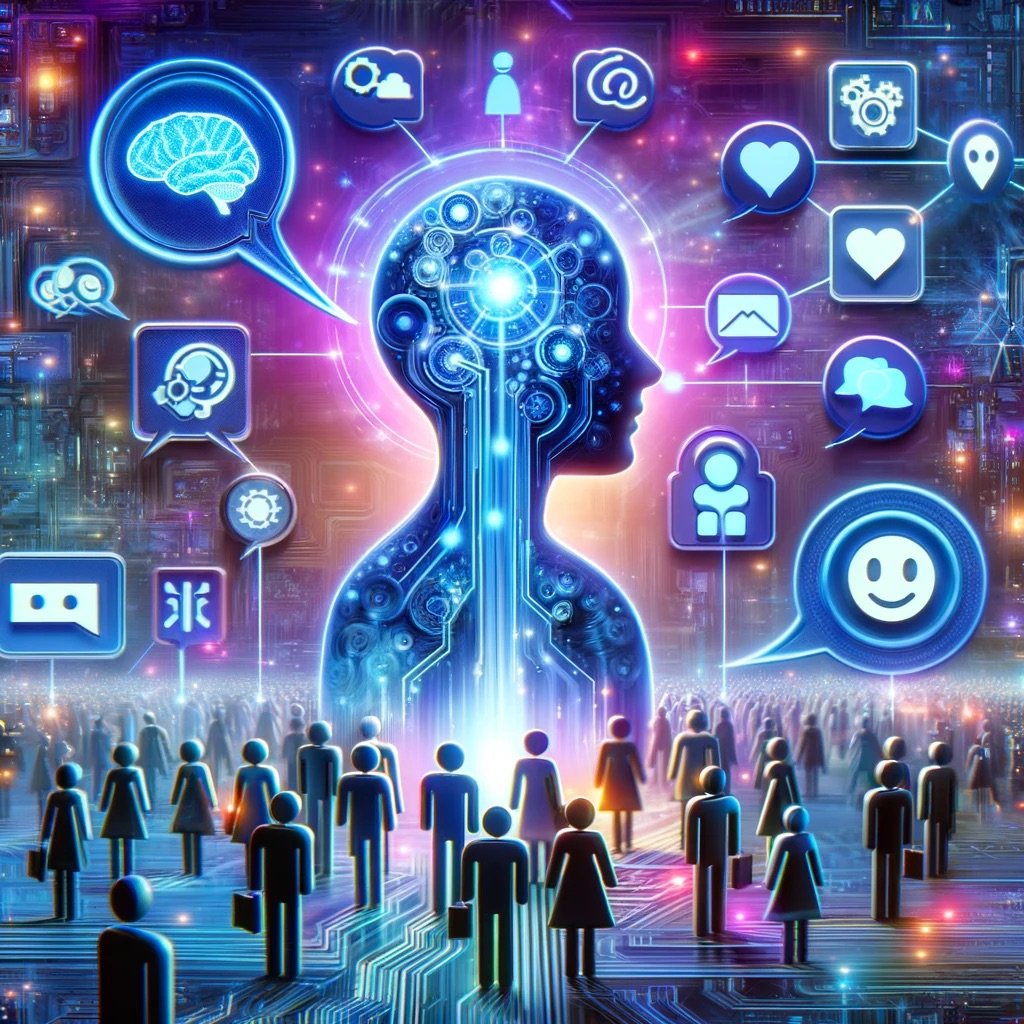
Using Artificial Intelligence to Improve Customer Satisfaction
Before jumping into the use cases of AI that can impact customer satisfaction, let’s take a quick look at two key strategy areas related to customers. Customer Success (CS) and Customer Experience (CX) are two critical concepts for modern business, both centered around optimizing the customer's interaction with a company, but they differ in their specific focus and execution.
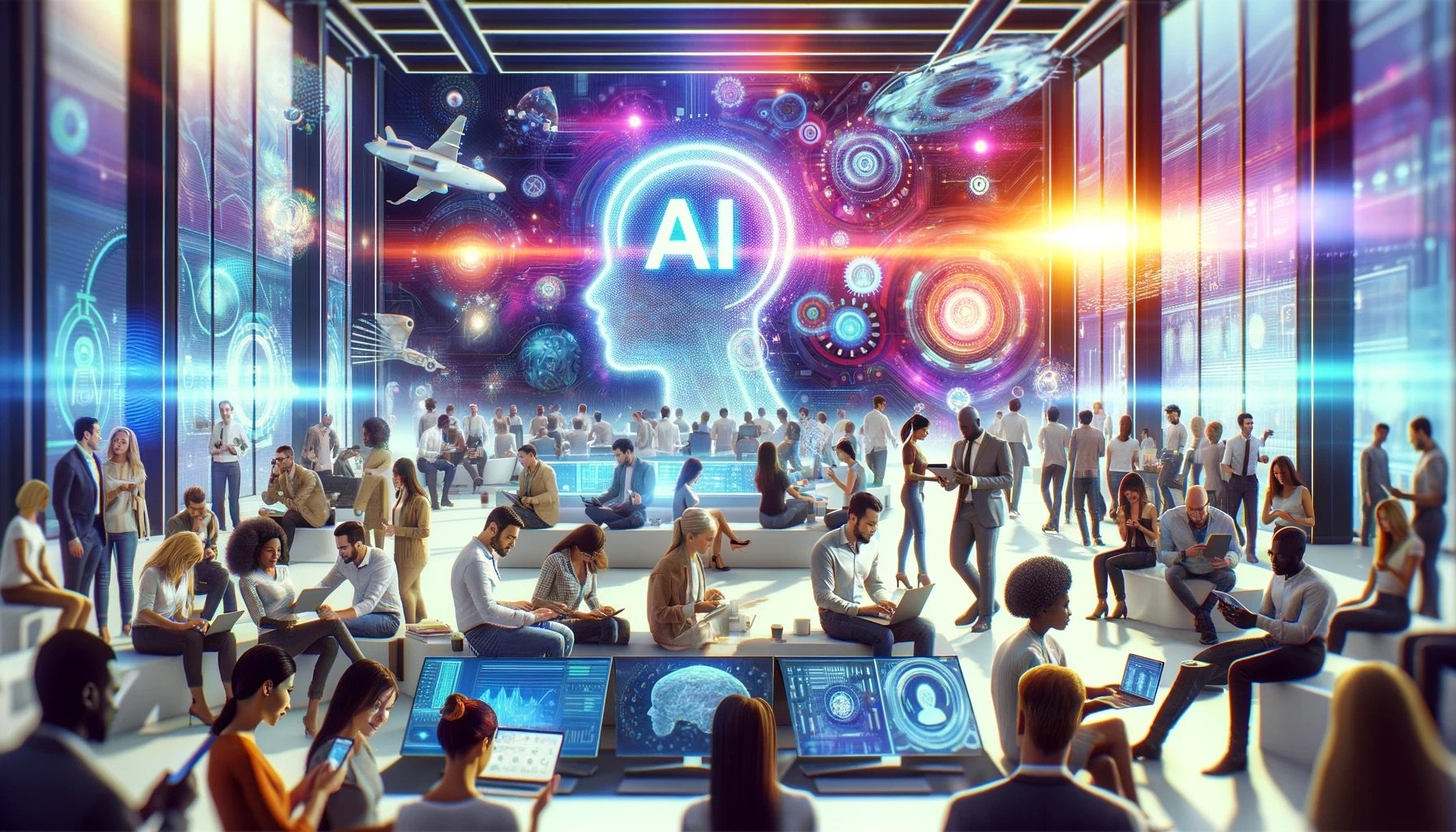
The Democratization of AI: A New Era of Accessibility and Integration
Artificial Intelligence (AI) is not new, it has been around for several decades in various forms, and even though its promise and potential was high, until recently its use has been relatively limited to process optimization, decision support, and enhancing customer experience. While those use cases are impactful, the number of users who either used the AI directly or used systems empowered with AI were limited. With the introduction and widespread access to generative AI tools like ChatGPT last year though, a rapidly growing number of people had the opportunity to use AI and realize / observe some of that potential benefit directly.
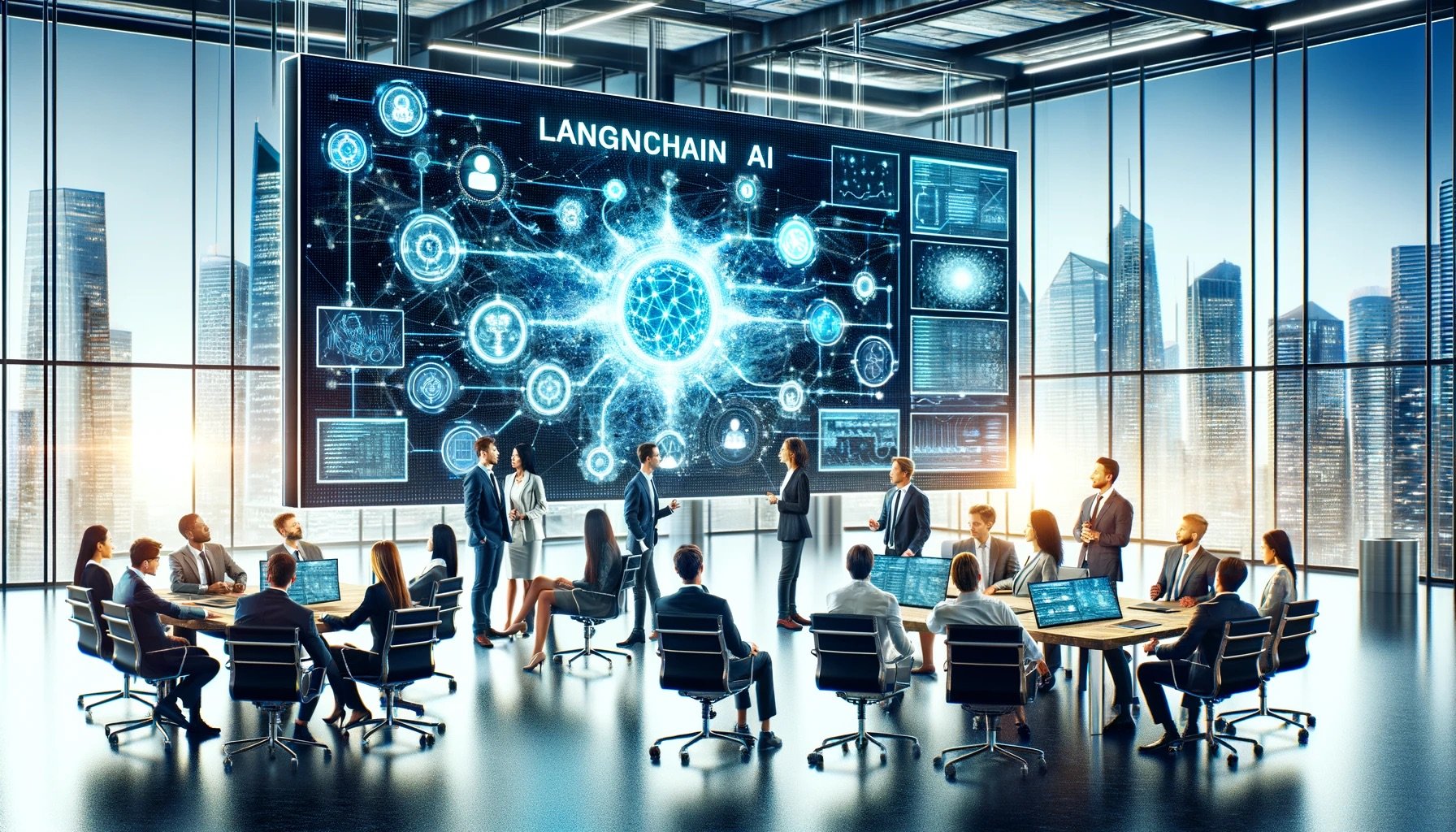
Using the LangChain Framework with Generative AI
LangChain is a framework designed for working with large language models (LLMs) like OpenAI's GPT-4. It's a set of tools and libraries that facilitate the development of applications built around LLMs. LangChain is designed to enable the chaining of LLMs with other tools and databases. It focuses on integrating various AI components, such as LLMs, databases, and search engines, to create more comprehensive and sophisticated AI systems. The idea is to leverage the strengths of different AI components to enhance the capabilities of language models in understanding and generating text.
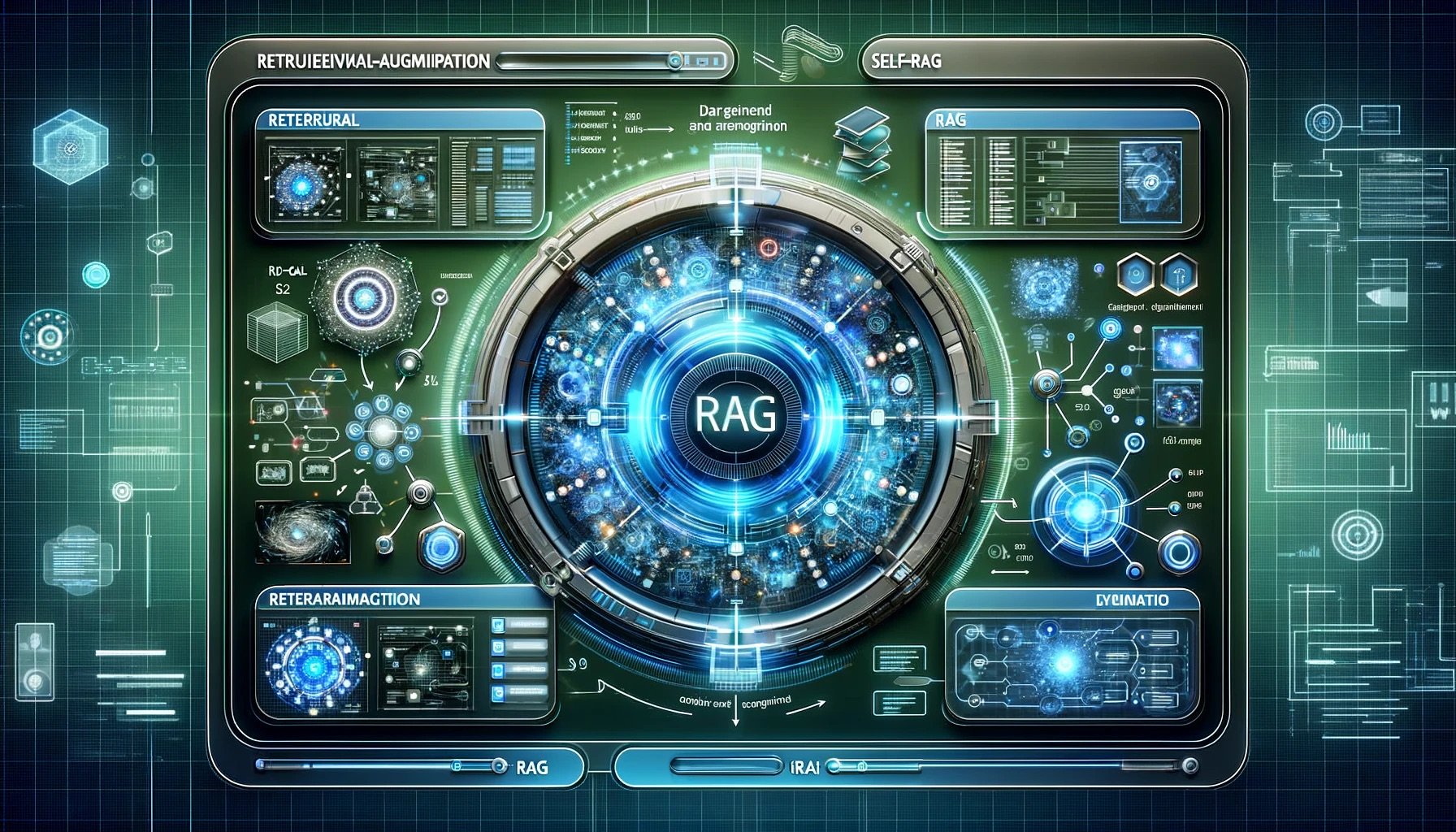
Retrieval-Augmented Generation
One of the most effective approaches to grounding large language models (LLMs) using the Data and Knowledge Base Integration method is Retrieval-Augmented Generation (RAG). RAG is an AI framework that retrieves facts from an external knowledge base to ground LLMs on the most accurate, up-to-date information.
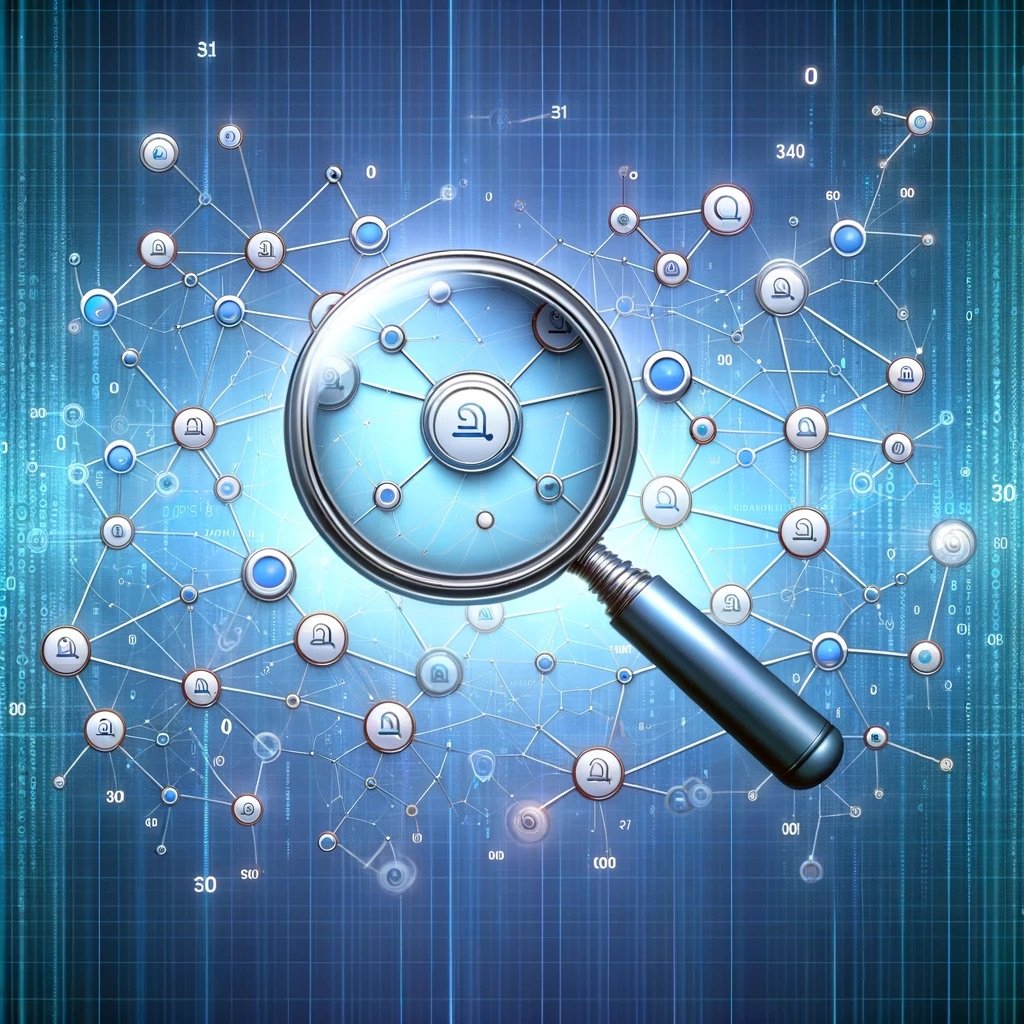
Understanding Semantic Search
Semantic search refers to the process of searching for information that understands the intent and contextual meaning of the search query, rather than just matching keywords. It involves interpreting the nuances of language, including synonyms, variance in word order, and the relationship between words, to provide more relevant and accurate search results.
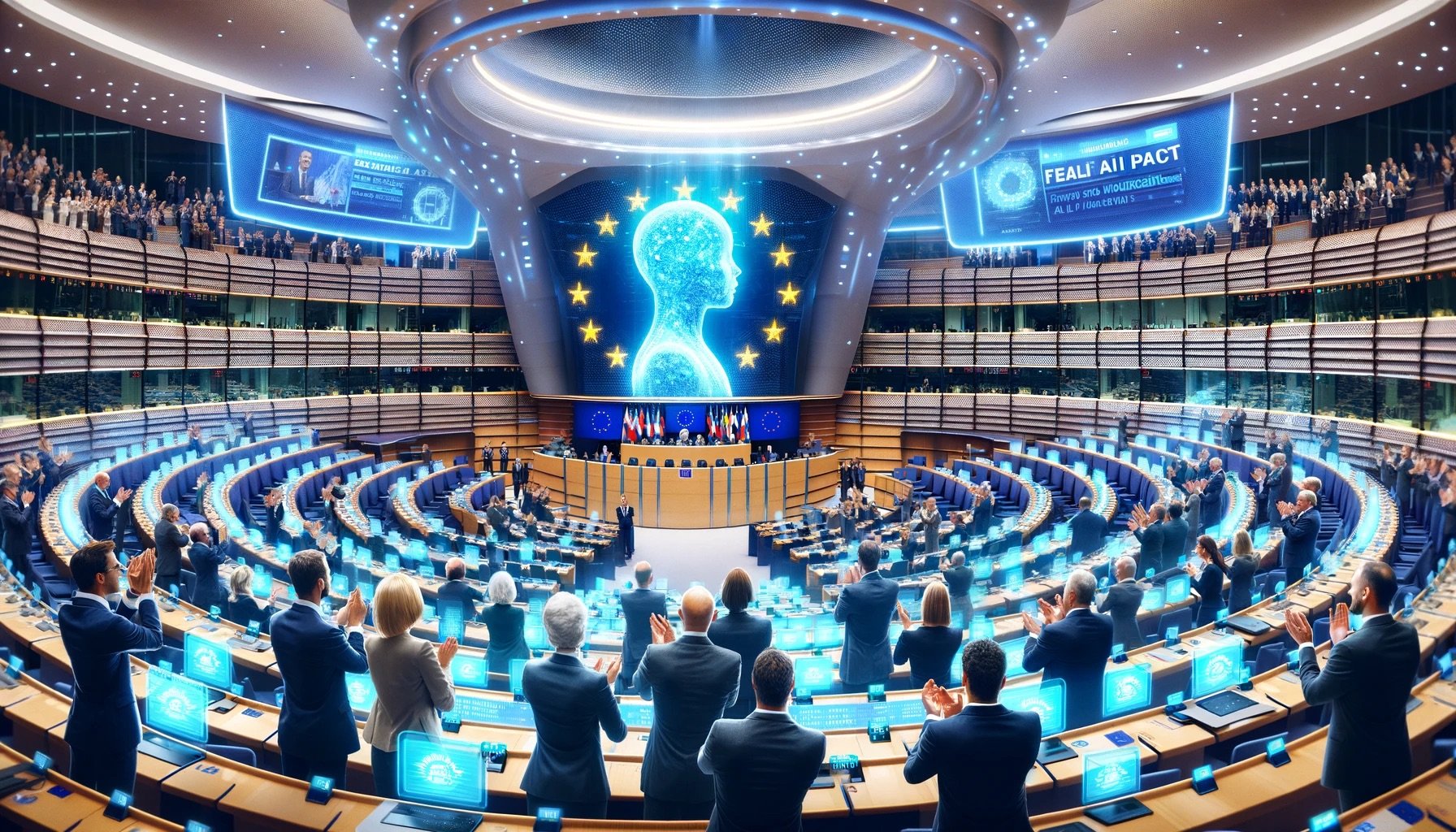
Reaching Agreement on the European Union’s AI Act
On December 9, 2023, the European Parliament and Council reached a provisional agreement on the European Union's Artificial Intelligence Act (EU AI Act), a comprehensive legal framework for AI. The Act aims to ensure that AI in Europe is safe, respects fundamental rights and democracy, and fosters an environment where businesses can innovate and expand. The legislation establishes obligations for AI systems based on their potential risks and impacts. It represents a significant step in regulating AI technologies.
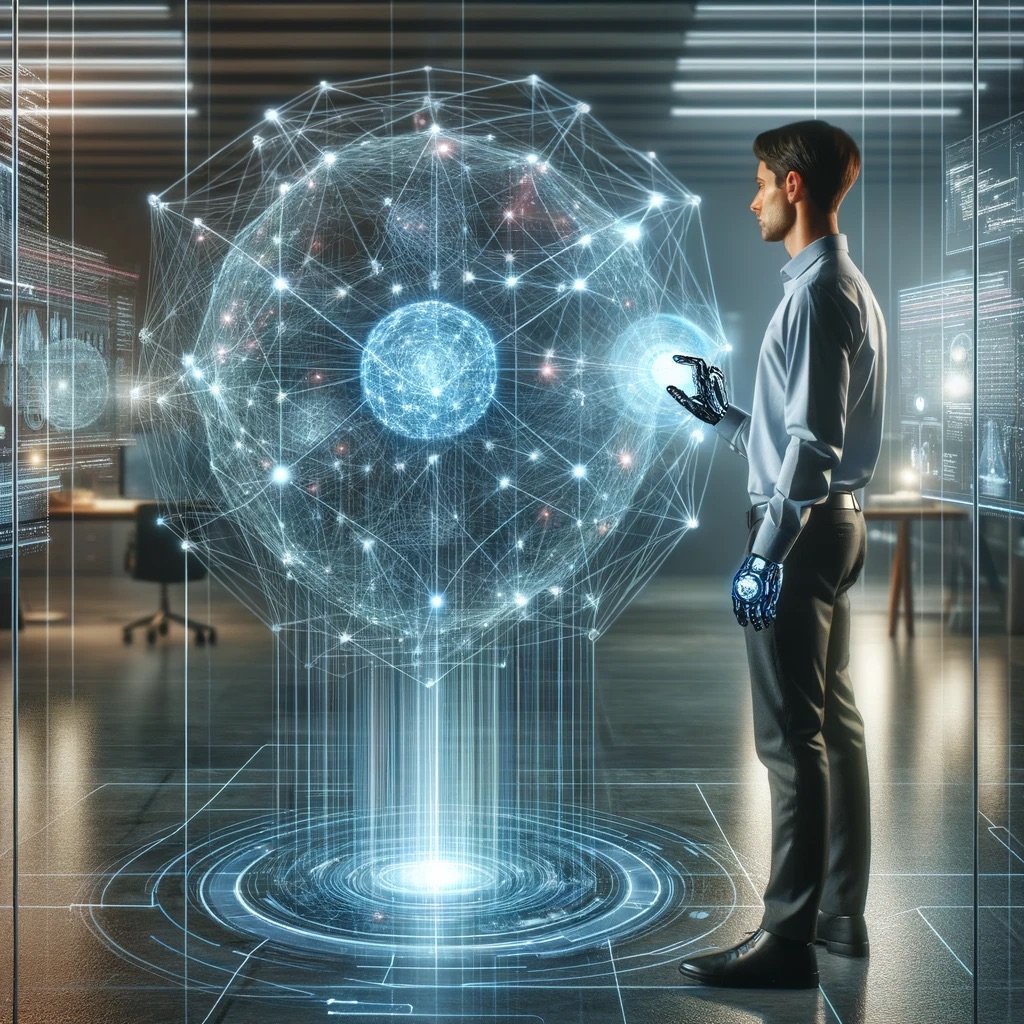
Understanding the Vector Database
A vector database is a type of database designed to efficiently store, index, and query vector data. Vector data, in this context, refers to arrays of numbers (vectors) that represent complex data types like images, sounds, texts, or any high-dimensional data. This database type is particularly relevant for machine learning and AI, where such data is commonplace.
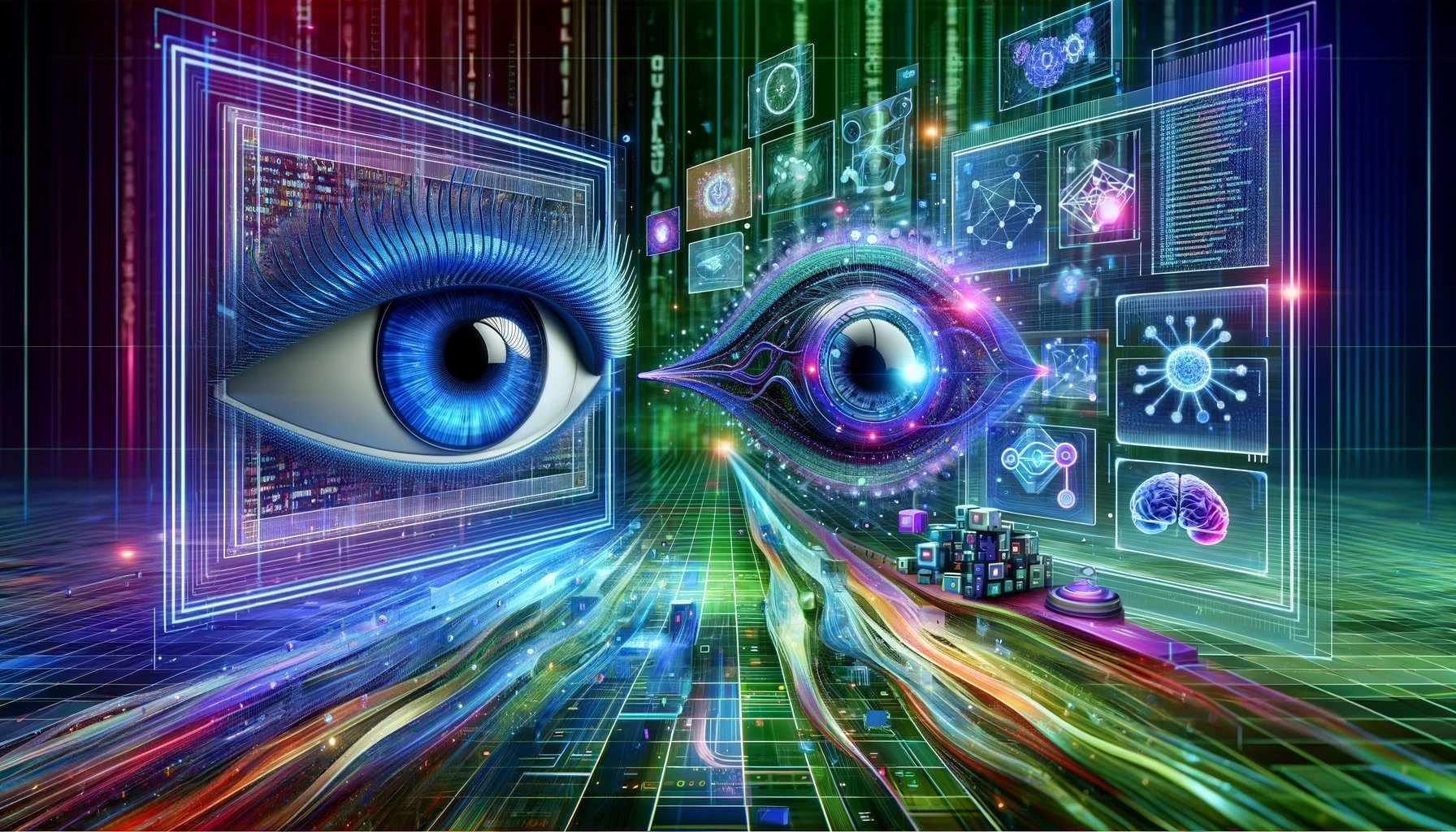
Computer Vision and Large Vision Models
Computer vision is an interdisciplinary field that deals with how computers can gain high-level understanding from digital images or videos. It attempts to automate tasks that the human visual system can do. Computer vision integrates aspects of image processing and computer science, and it employs methods from fields such as machine learning, pattern recognition, neural networks, and artificial intelligence.
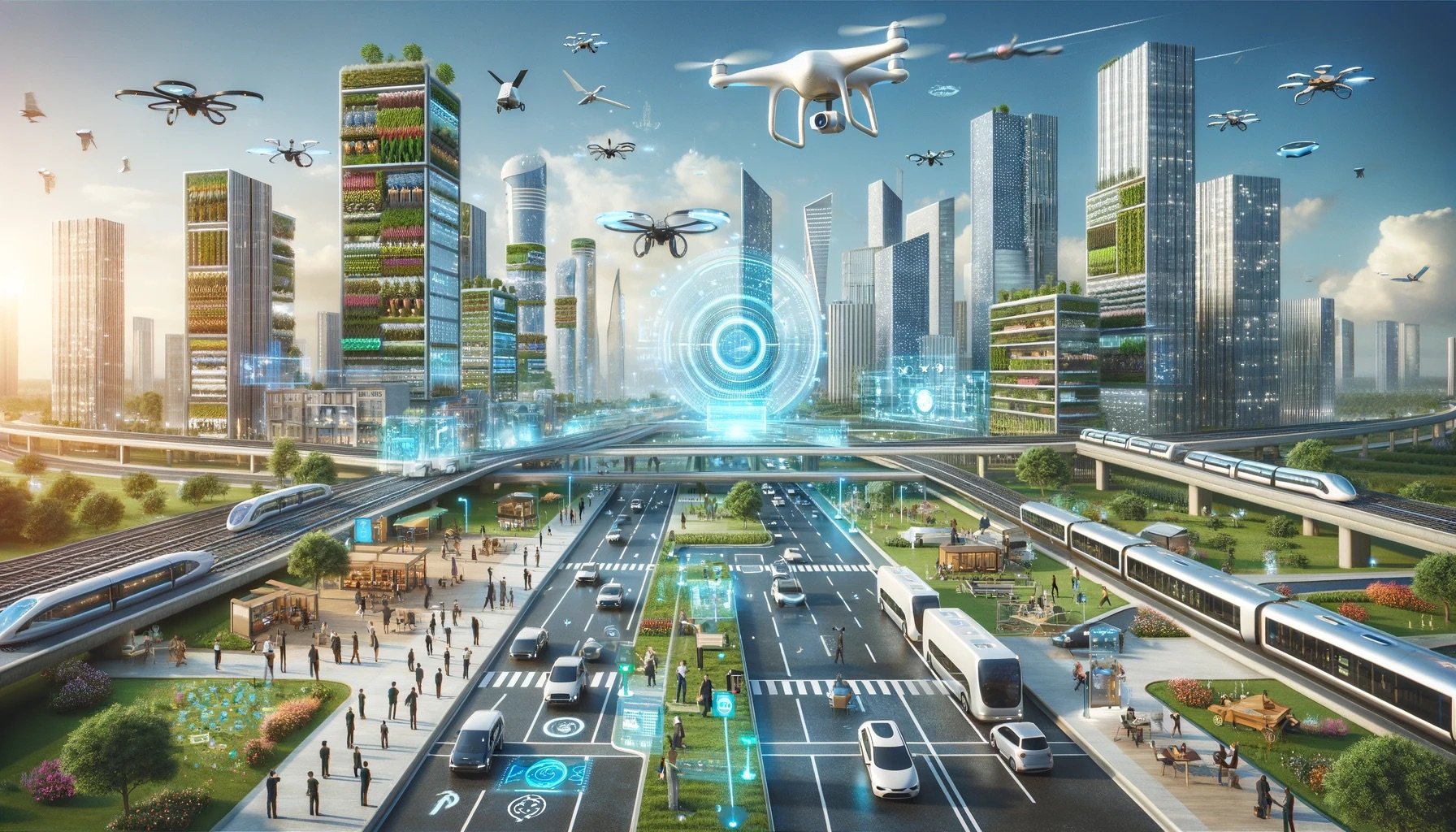
Five Trends for Emerging Technologies in 2024
There are a number of threads we could pull in emerging technologies, so boiling the list down to five is difficult. Of course this is my own limit, so I suppose there’s some argument to expand the list. I’ll resist that though, and try to focus these few trends on the most consequential in the areas in which I am currently focused; customer experience and CRM, artificial intelligence (AI) and adopting a digital first business strategy. Even with that focus I struggled to keep the list to five, but here’s where I landed:
AI Application Stores
Generative AI as the new platform
Quantum Computing + AI
Synthetic Customers
Large Vision Models (LVMs)
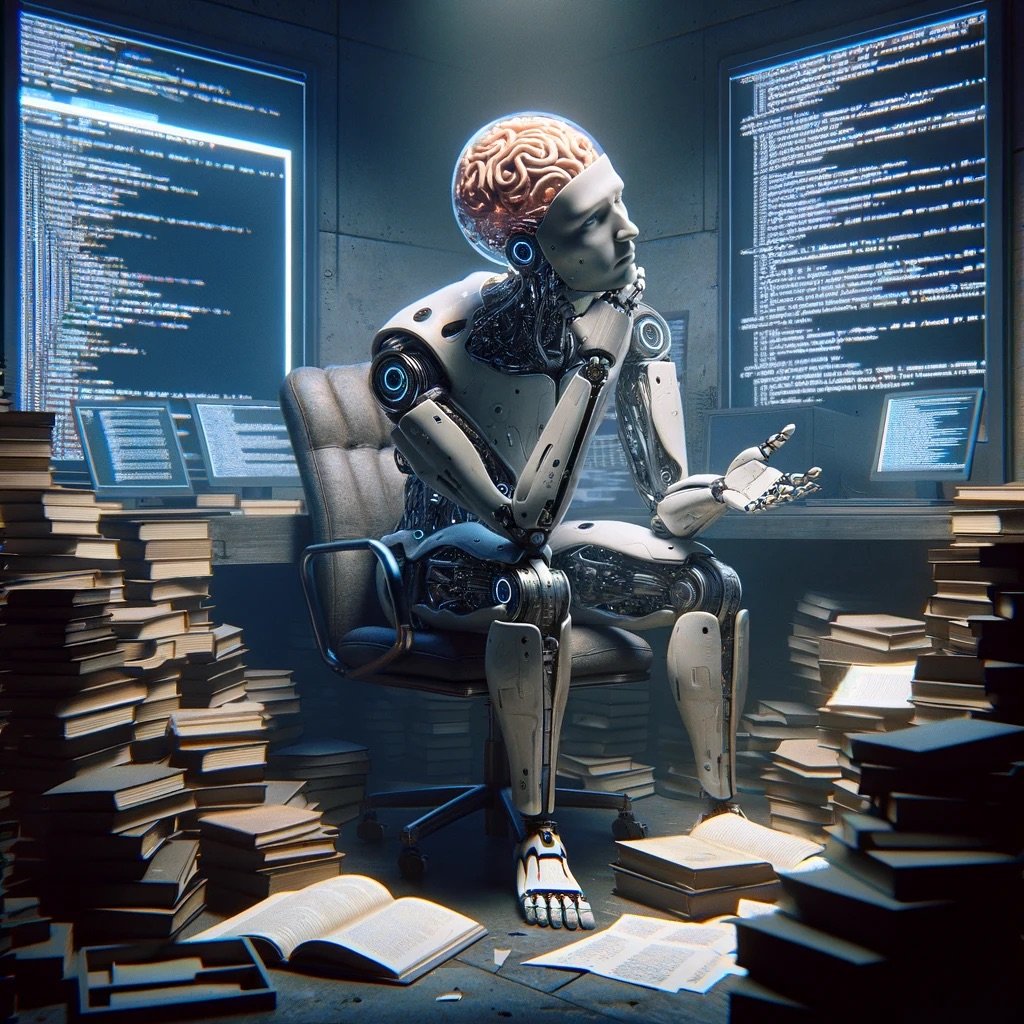
Understanding the Limitations and Challenges of Generative AI
It may seem unnecessary to provide an introduction to generative AI at this point, but just in case, let’s start there to level set; then dig into the limitations and challenges. Generative AI refers to a class of artificial intelligence that specializes in creating content, whether that be text, images, or even music. This technology operates by learning from massive datasets to generate new, original material that resembles the learned content. The most familiar examples include text-based models like ChatGPT, image generators such as DALL-E, and AI that composes music. While the potential of generative AI is significant, offering innovative solutions across various sectors including marketing, design, and entertainment, it is not without limitations and challenges.

Disambiguation: Generative AI for Higher Education and the Future of Work - TRANSCRIPT
The release of ChatGPT and other generative AI tools over the past year has caused many diverse reactions from higher educational institutions and educators. Many see opportunity and the need to make sure students are knowledgable about the technology, while others have pushed back, opposed and restricted the use of generative AI. Join us as we discuss the use of generative AI in higher education and its impact on the future of work with Keeley Meetze, CEO of the Keeley Company LLC.
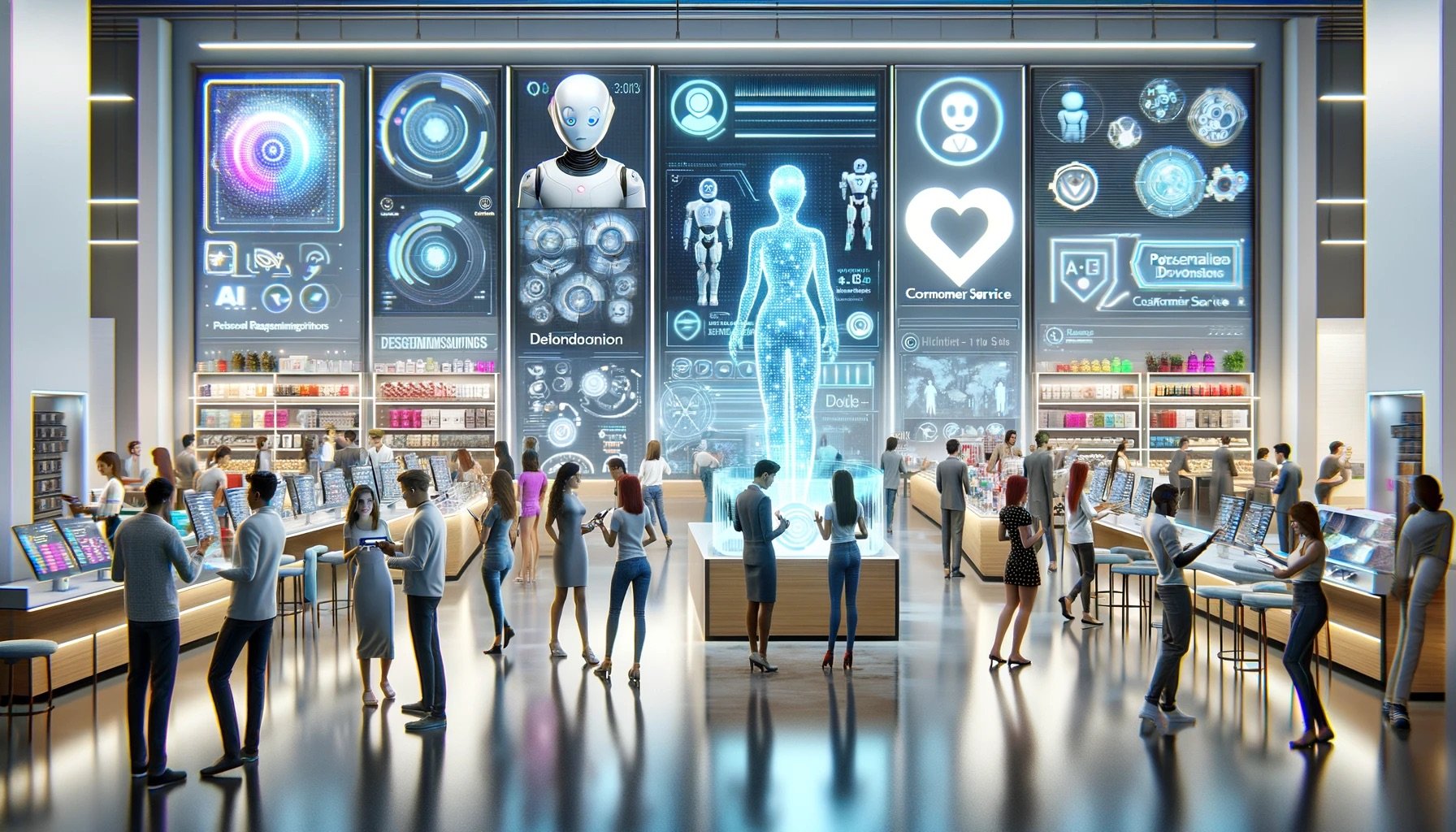
The Role of AI in Personalizing Customer Interactions
Personalizing customer interactions at scale is an ongoing goal for businesses, that has proven to be difficult and resource intensive. Artificial Intelligence (AI) has the capability to greatly simplify and improve personalization, offering businesses the ability to understand their customers better, customize recommendations, and ultimately make every customer feel seen and valued. Let’s explore the growing role of AI, including generative AI, in personalizing customer interactions, the techniques and processes involved, the desired outcomes, and the benefits for both the customer and the company.
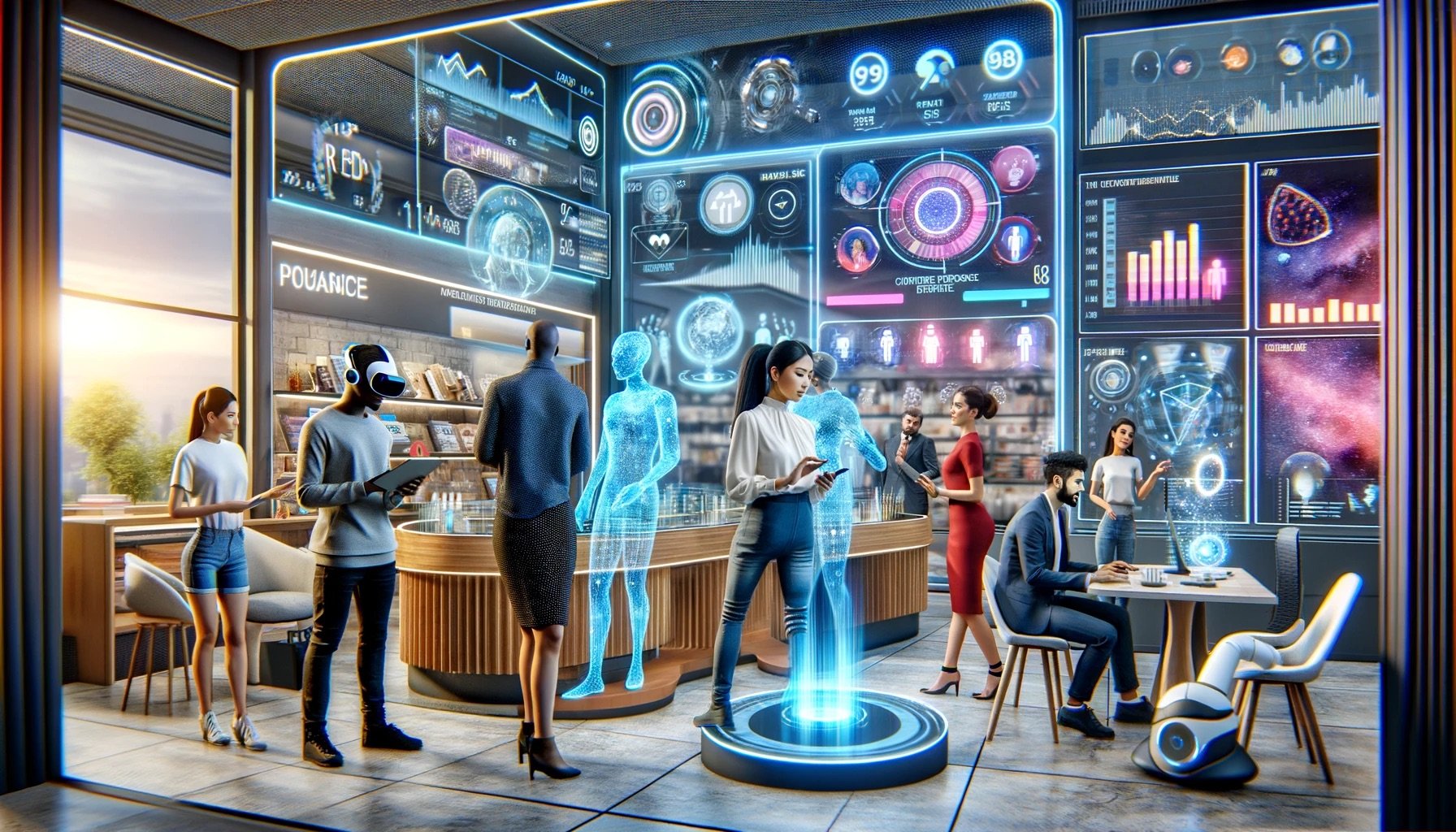
Customer Experience Trends for 2024
Customer experience (CX) is the cumulative perception and feelings a customer develops through their interactions with a company, encompassing every touchpoint from initial contact to post-purchase support. It's an all-encompassing journey that shapes the customer's view of the brand, influenced by the quality of customer service, ease of use, product satisfaction, and emotional connection. As businesses continue to evolve in 2024, understanding and enhancing customer experience has become a crucial element for success. This focus on CX reflects the growing recognition of its critical role in building brand loyalty, fostering positive word-of-mouth, and driving sustainable growth. In this context, exploring the latest trends in customer experience is vital for any business aiming to thrive in today's competitive marketplace.
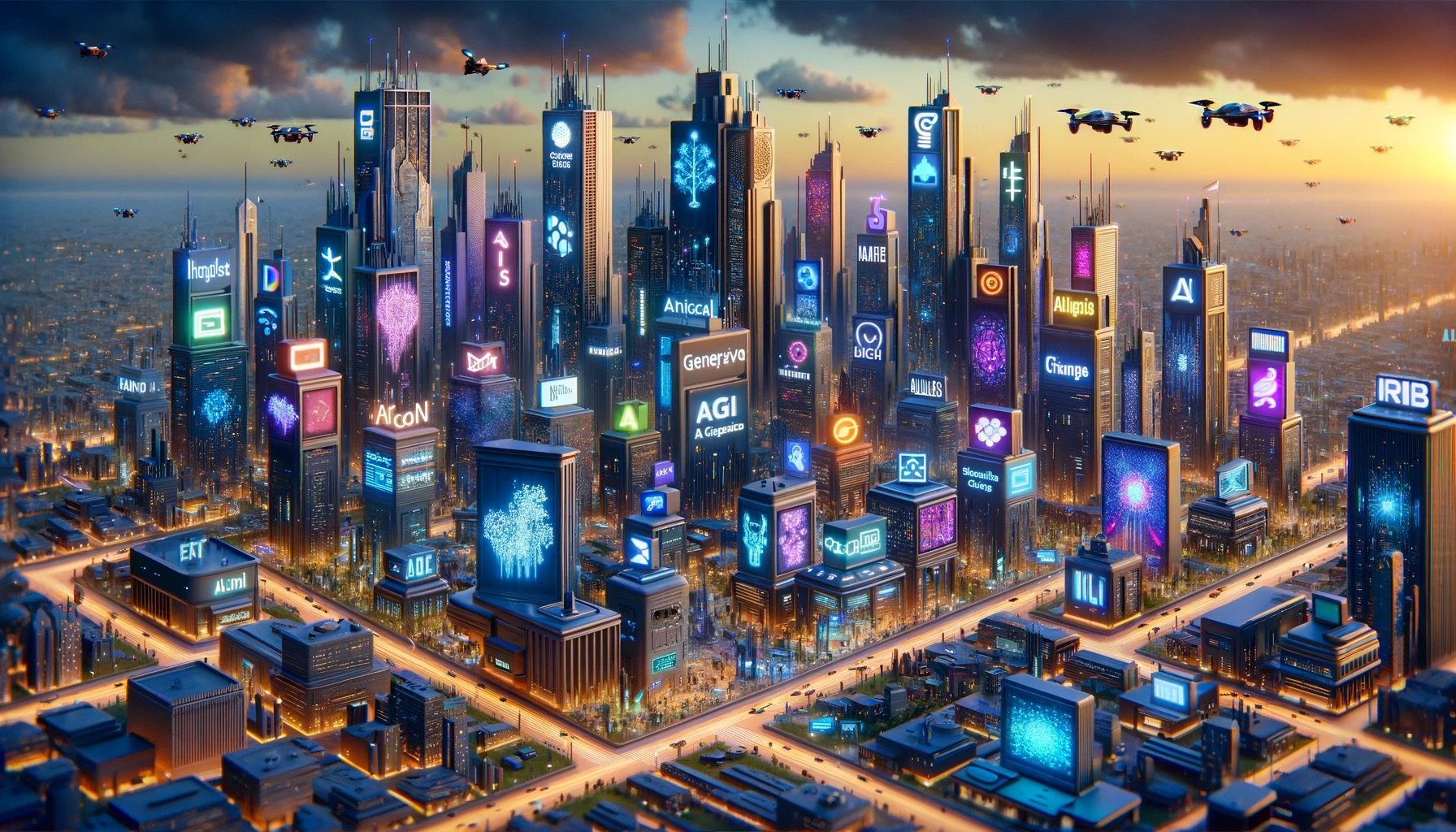
The OpenAI Debacle - UPDATED
I’ve debated writing something on the OpenAI drama since the first announcement on Friday. Everytime I’d start there would be a new development / announcement / rumor. Just keeping up was nearly a full time job. And the story isn’t over at this point (Tuesday). There is a long list of articles and blogs and linkedIn posts on the subject, so sharing something new and useful is a challenge. There are some lessons in this somewhere though, so I’ll try to pull out some useful observations.
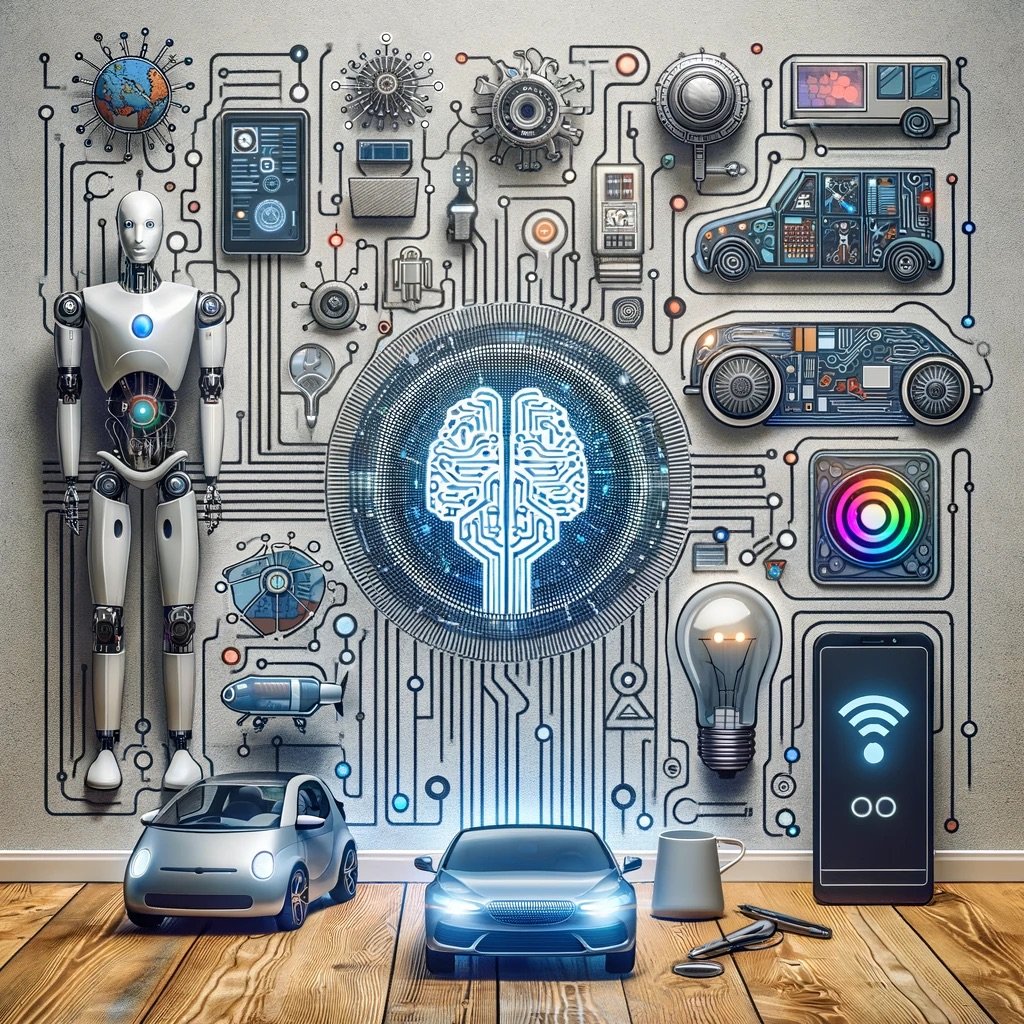
Artificial Intelligence: Beyond Generative AI
Over the past year the top tech topic has been generative AI. AI though, is not new, it has it roots back a few decades. While generative AI is a fairly recent capability, there are a number of other types that are currently in use across a variety of business use cases. Generative AI improves on some of those capabilities of course, but to really understand how a business can use AI to the best benefit, it’s helpful to have an understanding of the other types of AI, and how they can be used. The following graphic shows all the current AI types. It does not talk about future types though, like artificial general intelligence (AGI). While a very interesting topic, that’s a subject for another post.
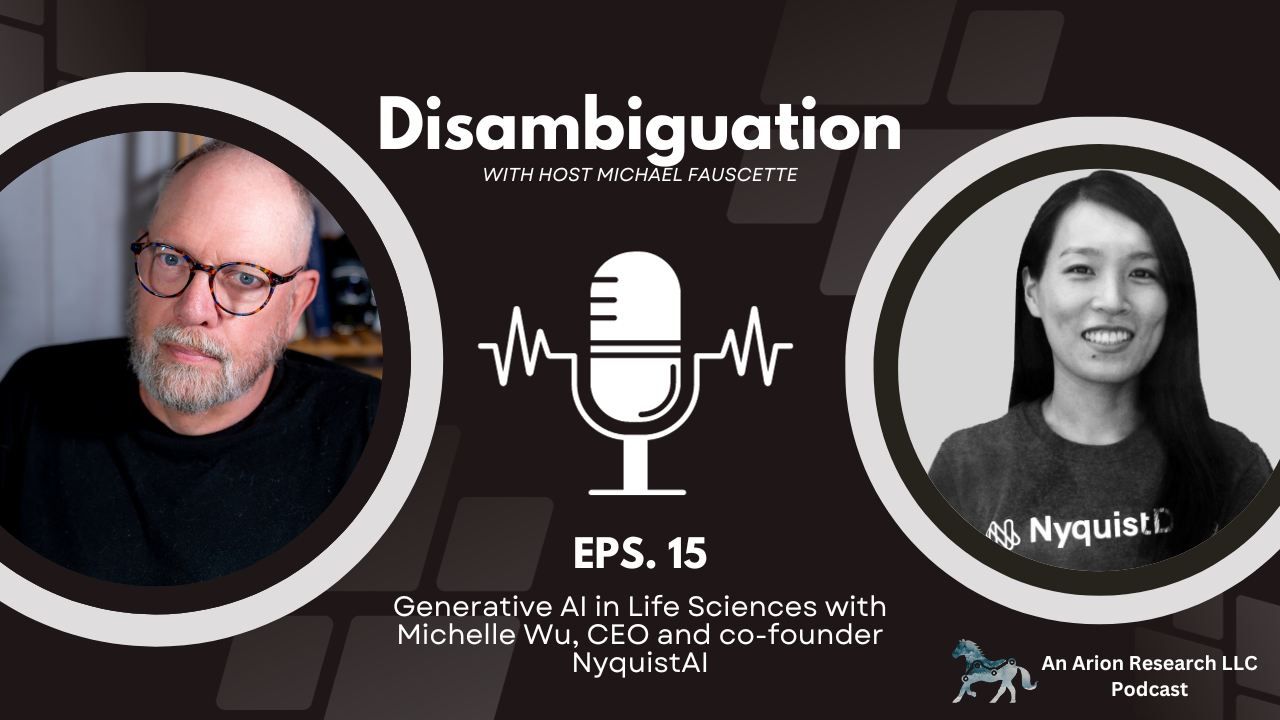
Disambiguation: Generative AI for Life Sciences - Transcript
Generative AI use is rapidly growing across many business functions, and generally is seen as a tool to boost the productivity of your teams. Productivity isn't the only benefit though, and companies are starting to look for generative AI platforms that can provide competitive advantage by addressing specific industry vertical use cases. I'm joined by Michelle Wu, CEO and co-founder of NyquistAI to explore the industry vertical use cases in life sciences. This episode is the first in an ongoing series to look at industry vertical use cases across a variety of industries.
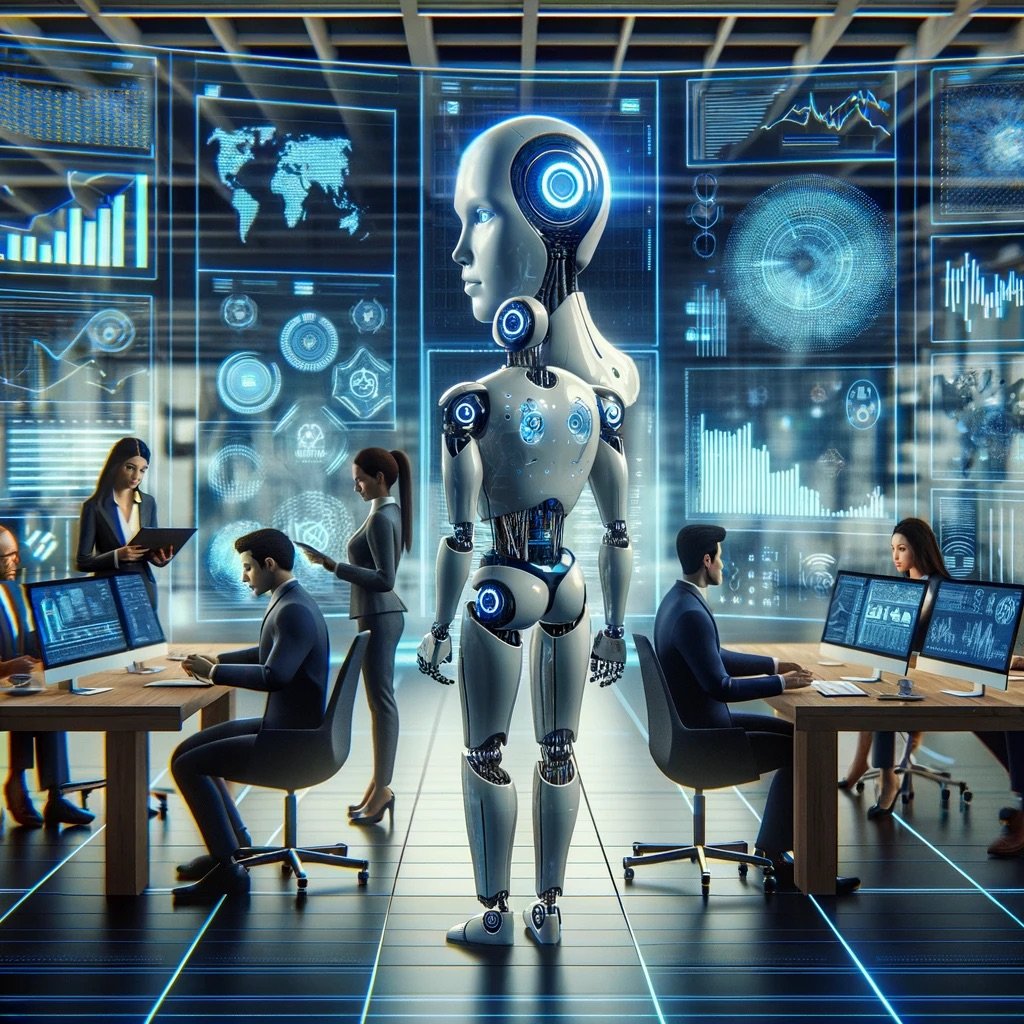
Generative AI for Finance and Accounting
Generative AI has the potential to revolutionize finance organizations by streamlining processes, enabling advanced data analysis, and providing valuable insights for strategic decision-making. The use cases, benefits, and challenges of implementing generative AI in the finance function are diverse. Applying generative AI enabled tools can impact the entire finance organization; automating mundane tasks, adding advanced / predictive analytics, improving and automating financial reporting and significantly enhancing risk management and mitigation.
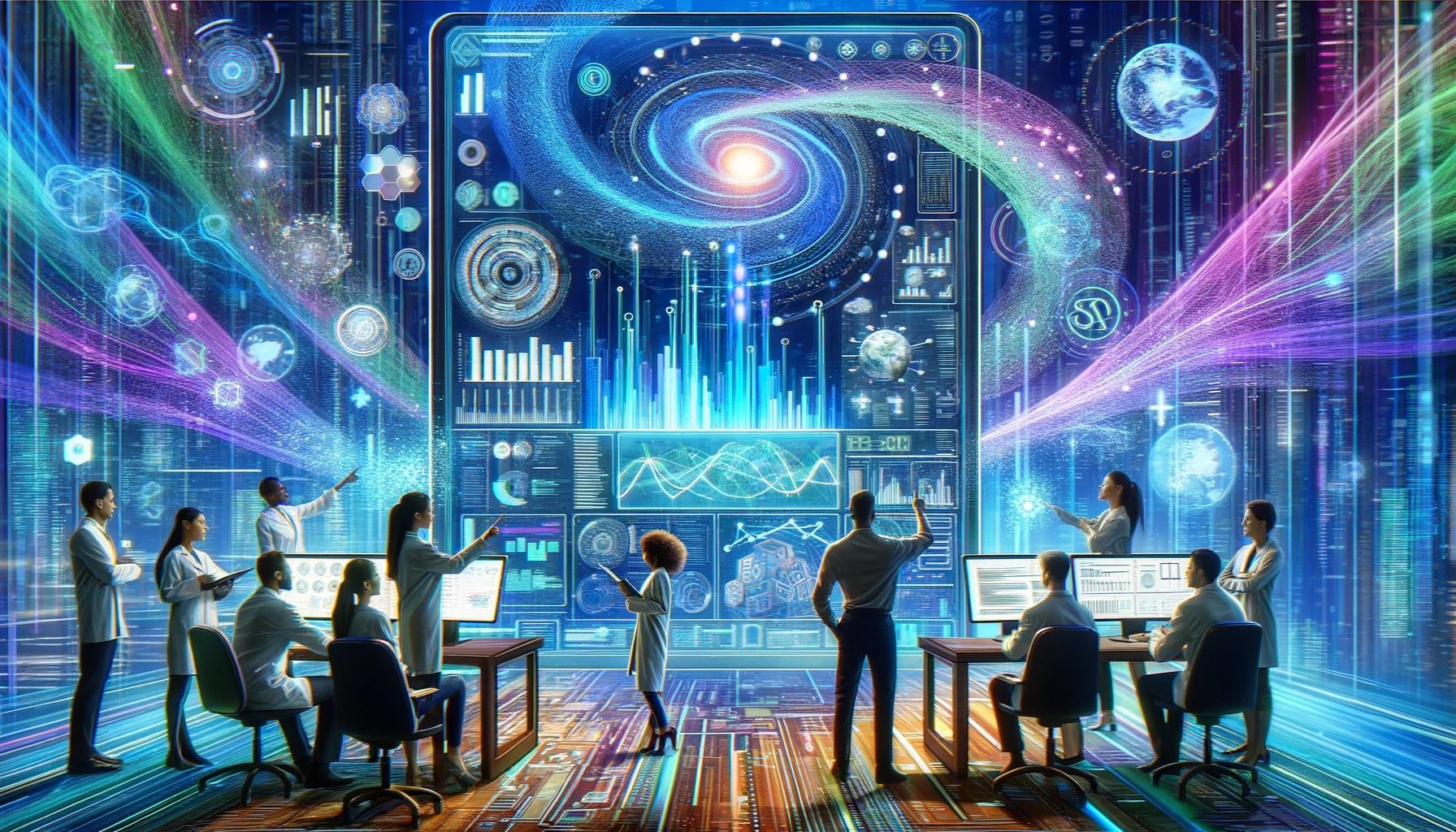
Synthetic Data
Synthetic data is artificially generated information that mimics real-world data. Unlike data collected from actual events or processes, it is created using algorithms and simulation techniques. This type of data can replicate various characteristics of genuine data, making it a valuable asset in situations where real data is scarce, sensitive, or difficult to obtain.
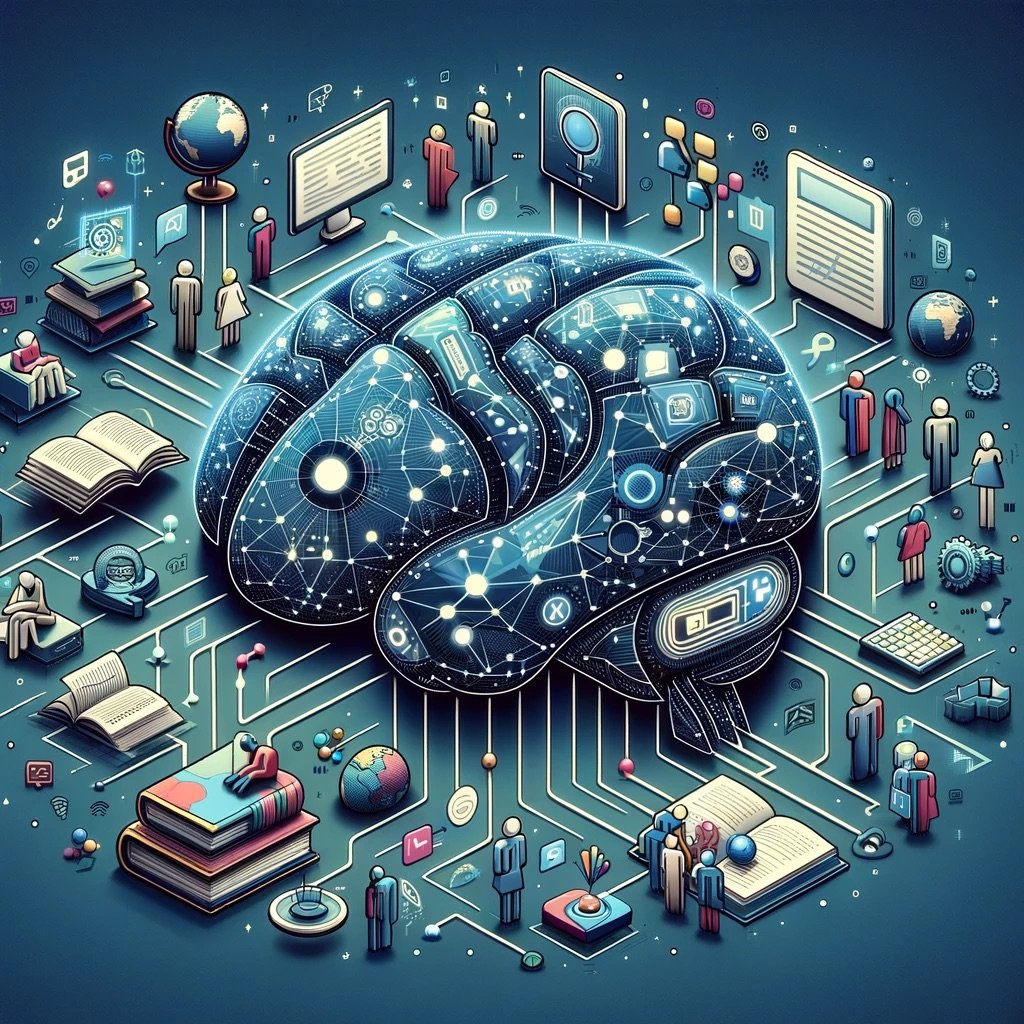
Grounding Large Language Models
Grounding helps to ensure that language models interact with users in a way that is informed, relevant, consistent, and trustworthy. The process of grounding large language models (LLMs) involves anchoring their responses in real-world knowledge and ensuring they maintain relevance to the context. Language models should be grounded in order to understand and relate to empirical data, avoid hallucinations, improve interaction with the physical world, enhance learning and adaptation, boost user trust, help deal with ethical considerations, and facilitate multimodal capabilities.

Disambiguation: AI and the Customer Journey - Transcript
AI and Generative AI have huge potential to help businesses improve the entire customer lifecycle. I'm joined by AI, CRM and process automation expert Jeff Nicholson for a wide ranging discussion on AI and generative AI in sales, marketing and customer service and how the application of these technologies can enhance the customer journey.